Can the Upper Midwest drive digital innovation in manufacturing and logistics?
Legacy Manufacturing Hubs
Manufacturing and farming ecosystems in the US and around the world have developed around a combination of the following ingredients: raw materials (minerals, crops), energy (electric, hydro, wind, solar), logistics (bays/ports, rivers, roads), talent/labor pools, and viable operating economics. A combination of investment capital, balance sheet leverage, and public policy has provided the needed financial backing to scale up.
In the US, several manufacturing ecosystems have come to exist in different regions over the decades, focusing on aerospace/avionics, automotive, food and farming, healthcare, metals and mining, oil and gas, and semiconductors/electronic components/systems. Upper Midwestern states are prominent in this list. These ecosystems have been using current-generation industrial/process controls systems, heavy equipment, and ERP systems.
Industry | Region | Anchor Companies |
Aerospace/Avionics | California | McDonnell Douglas, Hughes Aircraft. |
Kansas | Beechcraft, Cessna. | |
Washington | Boeing. | |
Automotive | Michigan, Indiana, Ohio | Chrysler, Ford, GM, Visteon. |
North Carolina | Borg Warner, Bridgestone, Caterpillar, Continental, Cooper, Daimler, Denso, Freightliner, Goodyear. | |
South Carolina | BMW, Mercedes, Bridgestone, Volvo, Magna. | |
Food and farming | Central Valley, California | Del Monte, Dole. |
Wine Country, California | Gallo, Mondavi, Jackson, J Lohr, Korbel, Rutherford, Sebastiani, Sutter, Wente. | |
Minnesota | Cargill, General Mills, Land O’Lakes, Hormel Foods. | |
Missouri | Monsanto. | |
Wisconsin | Schreiber Foods. | |
Iowa | Rembrandt, Burke, West Liberty. | |
Idaho | Simplot. | |
Healthcare | Minnesota | Boston Scientific, Mayo, Medtronic. |
Illinois | Abbott Labs. | |
Industrial Equipment | Illinois | Caterpillar. |
Iowa | John Deere, Vermeer. | |
Minnesota | Honeywell. | |
Wisconsin | Rockwell Automation. | |
Metals | Pennsylvania, Ohio | Bethlehem Steel, U S Steel, USX. |
Mining and materials | Minnesota | 3M. |
Oil and gas | New Jersey | Arco. |
Texas | Exxon-Mobil, Schlumberger, Valero. | |
Paper | Idaho | Boise-Cascade. |
Semi-conductors | Silicon Valley | ICs – Broadcom, Intel, nVidia, Marvell, SanDisk/Western Digital. IC manufacturing equipment – Applied Materials, LAM Research. |
San Diego | ICs – Qualcomm. | |
Idaho | ICs – Micron. |
Table 1: US regions, manufacturing ecosystems, and key anchor companies.
Digital Manufacturing – Requirements
In the age of ‘traditional’ manufacturing, the resources needed included:
- Skilled labor.
- Power (electricity, coal, hydro/wind/solar).
- Raw materials, natural resources (e.g., farm products, ore, water).
- Railroad, shipping, air transport.
In the age of digital manufacturing, from design to shipping, the following capabilities are important:
- Internet infrastructure.
- Rapid prototyping.
- Automation – robotics, self-navigating vehicles.
- End-to-end integration with supply chain and logistic partners for real-time visibility.
- Advanced STEM professionals in computer science, materials science, and life sciences (clinical medicine, plant biology, molecular biology, and biochemistry).
- Digitally-literate labor with skills (e.g., workers who can operate analytic software or fix a clean room air shower to maintain low ppm particle levels).
- Predictive analytics for preventive maintenance, resource management, etc.
Advanced technologies useful for these capabilities include:
- IoT (e.g., for discrete automation, process controls, asset tracking, robotics, infrastructure management).
- Machine learning (e.g., for instrumentation & control).
- New materials, including nanomaterials.
- 3D printing for prototyping, small-batch quick-turn manufacturing, and on-demand inventory.
- Secure technologies such as block-chain (e.g., for transactions, contracts, logistics).
- AI/predictive analytics (e.g., for monitoring and control, preventive maintenance, ERP).
Next-generation digital manufacturing initiatives have taken root in regions where current manufacturing ecosystems have existed. For example, Tesla’s California factory is housed in the earlier NUUMI (General Motors and Toyota) plant in Fremont in Silicon Valley, where the plant was originally set up in 1960 by General Motors due to cheap land, the port of Oakland nearby, and a freight railroad along the Bay. Similarly, SpaceX (Hawthorne, CA) has leveraged design and manufacturing resources originally set up by McDonnell Douglas and Hughes Aircraft in the Los Angeles area near the port of Long Beach.
The Upper Midwest
Considering the extraordinary value creation of companies such as Tesla and SpaceX, there is a significant investment thesis in enabling next-generation manufacturing organizations to arise in the Upper Midwest, where many similar current-generation manufacturing ecosystems already exist.
Given the democratization (i.e., global availability) of some of the new technologies, the presence of global companies such as Google, Amazon, and IBM in most of the major cities in the Upper Midwest, and the significant academic centers across the region; highly-trained resources are available throughout the region to enable advanced digital manufacturing centers of excellence.
The Upper Midwest also has strong attributes to attract and retain relevant labor pools. Table 2 shows the relative desirability of different regions in the US as destinations for STEM workers. The statistics for the Upper Midwest, broadly including the greater Cincinnati, Columbus, Chicago, Denver, Madison, Minneapolis/St. Paul, and Pittsburgh areas, speak for themselves.
Rank (1=Best) | Metro Area | Total Score | Prof Opptys | STEM Friendliness | Quality of Life |
1 | Seattle, WA | 73.60 | 2 | 4 | 15 |
2 | Boston, MA | 71.94 | 7 | 1 | 43 |
3 | Pittsburgh, PA | 65.90 | 12 | 11 | 9 |
4 | Austin, TX | 65.15 | 6 | 8 | 27 |
5 | Minneapolis/ St. Paul, MN | 64.95 | 19 | 6 | 17 |
6 | Madison, WI | 64.00 | 13 | 16 | 13 |
7 | Salt Lake City, UT | 62.96 | 9 | 14 | 18 |
8 | Springfield, MA | 62.80 | 36 | 2 | 7 |
9 | Chicago, IL | 60.71 | 49 | 13 | 8 |
10 | Atlanta, GA | 60.69 | 5 | 27 | 31 |
11 | Cincinnati, OH | 60.51 | 16 | 33 | 14 |
12 | San Francisco Bay Area, CA | 60.50 | 3 | 7 | 67 |
13 | Columbus, OH | 59.71 | 33 | 21 | 21 |
14 | Denver, CO | 57.73 | 10 | 24 | 37 |
15 | San Diego, CA | 57.39 | 59 | 23 | 16 |
16 | Sacramento, CA | 57.20 | 44 | 20 | 25 |
17 | Colorado Springs, CO | 57.00 | 17 | 54 | 20 |
18 | Worcester, MA | 56.88 | 43 | 3 | 65 |
19 | Richmond, VA | 56.58 | 8 | 32 | 44 |
20 | San Jose, CA | 55.79 | 18 | 18 | 53 |
Table 2: Desirability of cities in the US for STEM professionals. (Data Source: Richie Bernardo/ WalletHub, “2018’s Best & Worst Metro Areas for STEM Professionals”).
Superimposed with Table 1, Table 2 shows the opportunity for these regions to become magnets for next-generation manufacturing.
What Do the Next-Generation Manufacturing Innovations Look Like?
As a result, disruptive new companies are emerging in these regions, creating the investment opportunity to fund them from early-stage growth to scale-up. These companies are working with the incumbents shown in Table 1 to accelerate their evolution towards advanced digital manufacturing.
Examples of innovative companies focused on manufacturing and transportation in the upper Midwest include:
Proto Labs[i]– Rapid prototyping: allowing quick turnaround manufacturing.
FactoryFix[ii]– Contingent labor for equipment: predictive diagnosis for timely repair with skilled labor and relevant spare parts for better customer experience at lower cost.
Basin Commerce– River transportation: harnessing of shipping capacity on lake and river waterways using barge booking software.
Rambl[iii]- AI: sales call CRM logging and intelligence that analyzes calls for specific criteria and actions to surface insight.
Stemonix– Drug discovery: tissue manufacturing for testing potential side-effects of new drugs for neurological and cardiac diseases.
Aker– Crop management: Use of drones for crop management based on weather databases and image-based diseases detection.
The Four Legs of the Innovation Stool
Infrastructure, technology, labor, and capital are the four legs of the innovation stool. The Upper Midwest has all of these.
Through new digital manufacturing technologies focused on topline and bottomline benefits, today’s manufacturing ecosystems are ripe for significant new value creation.
At Great North Labs, we are focused on providing early-stage capital to innovators in the Upper Midwest, with an emphasis on next-generation manufacturing. We leverage partners and advisors with market and operating experience in manufacturing. We invest in, and provide guidance and advisory resources to technology startups like FactoryFix that are poised to disrupt the traditional manufacturing and logistics industries in the Midwest.
[i] Donald Krantz, Director at Proto Labs, is an advisor to Great North Labs
[ii] FactoryFix is a Great North Labs portfolio company
[iii] Rambl’s co-founders, Mitch Coopet and Brian Bispala, are advisors to Great North Labs
IoT and Analytics – Organizing the Industrial Internet
Figure 1: The third revolution: IoT and Analytics. [Image credit: General Electric]
The Evolution of IoT – Where we Came From
The first generation of IoT systems (IoT 1.0) was built mostly with data collected from IP-based sensors by monitoring applications. Whether standalone or embedded in phones, low-cost sensors, compact packaging and distributed power enabled new endpoints and systems. These monitoring applications served needs such as asset tracking, fitness monitoring, mood lighting, physical security, and others.
The second generation (IoT 2.0) leveraged the capabilities of infrastructure tools such as edge gateways, publish-subscribe buses, data warehouses, and API-based integration. The edge gateways allowed IP network segments to connect to sensor bus segments using a diverse set of protocols (e.g., RS-422, RS-485, BACnet, CAN, Fieldbus, Hart, LonWorks, Profibus, Seriplex, Zigbee, Z-wave, and others). The gateways extended the reach of these IoT systems across the many incumbent protocols and enabled the integration of the IP segments with legacy systems. The publish-subscribe buses made data-driven software architectures easier to implement and scale. The data warehouses enabled the integration of structured, semi-structured and unstructured data. The integration APIs enabled ingestion of data at scale. Together, these new building blocks enabled larger-scale IoT applications such as home monitoring, smart metering, power grid management, parking systems, next-generation environmental controls in buildings, windmill farms, warehouse management, etc., with varying degrees of commercial success based on the benefit provided vs. the insertion economics of each use case.
Today’s Frontier
With the larger data sets enabled by frameworks such as Hadoop and big data software such as Pivotal, the third generation of IoT systems (IoT 3.0) is integrating analytics for decision-making. These analytic platforms enable the processing and visualization of the IoT data sets. The large data sets and analytic tools identify aberrations with higher levels of confidence (statistical power) and detect ‘signals’ not seen before, they have lower detection thresholds, greater measurement sensitivity, and higher accuracy.
Applications based on these capabilities range from physical security for homes, buildings, and warehouses; to detection of diseases like lung disease, cancer metastases, or cardiac arrhythmias (see the Mayo Clinic and AliveCor’s recent work); and complex chemical analysis as in rare earth element detection. The availability of computing platforms at the ‘edge’ (e.g., gateways) enables distributed/local analysis.
“The Internet of Things is giving rise to a tsunami of data,” said Great North Labs advisor Ben Edwards (founding team member of home automation pioneer SmartThings). “The billions of residential sensors in people’s homes and the personal sensors on their bodies are sources of data of value to each of us, and depending on what we make available to others, to family members for our safety and well-being, to the retailers we buy from, to the health practitioners who take care of us.”
The proliferation of machine learning algorithms with new programming environments such as Python and dataflow libraries such as TensorFlow has opened up a wide range of new applications. These include anomaly-based security alerts, health and fitness monitoring, genomic analysis and biomarker detection for disease prediction, drones, and self-driving cars.
The addition of machine learning libraries to established platforms such as Matlab, R, SAS, and SPSS, is enabling insertion of machine learning into legacy applications.
The availability of these tools in public and private clouds has made their accessibility and deployment even easier.
Together, with supervised and unsupervised learning, the machine learning software is processing data sets with high data dimensionality, like those from mining, voice processing, drone navigation, and self-driving cars.
The integration platforms and IP-based communication are also enabling the integration of the IoT world with the enterprise world, making applications possible across hybrid computing and control environments such as airports, buildings, cargo ships, factories, hospitals, refineries and oil rigs. While this creates security issues for the enterprise as well as control systems, solutions such as micro-segmentation of hybrid systems are beginning to emerge.
Tomorrow – The New Startups
With products from companies such as Nvidia, Intel, Qualcomm, Broadcom, and now Google, real-time computing power is becoming available at the edge. With easier integration and low cost, it is becoming embeddable at sensing endpoints for applications such as drones, self-driving cars and trucks, personal walking/talking robots, personal assistants, point-of-care diagnosis, no-POS retail, smart logistics, and smart city applications from parking lots to secure airports and intelligent highways.
Adoption Outlook
Beyond analytics and monitoring, this fourth generation of IoT systems will be able to use analytics and machine learning for controls.
What is the outlook for the adoption of these applications? The answer is: it depends. And it is best found through analogies.
How confident do today’s chess masters or masters of the game of Go today feel betting against the machine? IBM’s Deep Blue computer beat chess champion Garry Kasparov in 1997. And as Great North Labs advisor Mitch Coopet (CEO of AI-focused Aftercode) points out, “Since 2016, Google’s Alpha Go platform has won against several Go masters using improved deep learning techniques.”
Or, when will the day come when your x-ray machine will have better diagnostic accuracy than your radiologist? Ahem, that day is already here.
Or, when will Alexa be able to detect tonal infection to assess mood? Based on indications from Amazon and makers of social robots and AI assistants, sentiment analysis will progressively improve the way machines will interact with humans.
Or, when will we be comfortable with self-driven cars? Completely autonomous navigation in 5-7 years may be unlikely, but it is equally likely that in 20 years, self-navigation will become a required safety feature for new cars.
Given the range of answers above, it is not a matter of if, but when, that real-time control using machine learning will be common. These systems will be able to handle use cases as diverse as (i) detecting rare earth minerals to help navigate the earthmoving equipment towards richer ore in a mining operation, (ii) making real-time sweeps at airports to pinpoint explosives across large masses of people, luggage, and infrastructure, (iii) ensuring that the robots deployed in automotive assembly stay within the extremely tight tolerances of frame construction, and (iv) predicting the failure of a component in a high value CT scanner or remote ATM to dispatch the skilled repairman in a timely way to avoid downtime (a business that Great North Labs has invested in).
The Innovation Ecosystem of the Industrial Internet
“Business Insider projects that there will be 55 billion IoT devices operating in the world by 2025, impacting a broad set of industries including automotive, consumer products, electronics, medical devices, and industrial equipment,” notes Great North Labs advisor Robert Bodor (Vice-President and GM, Americas, at Protolabs).
At Great North Labs, with an ambitious vision, we aim to help build the innovation ecosystem of the Industrial Internet visualized by IoT 3.0. This is because we believe the ingredients to build it are uniquely within reach for us.
The three pillars of any tech-enabled disruption are entrepreneurs/developers, adopters/enablers, and capital.
- Entrepreneurs/developers. The Upper Midwest created the industrial enterprise. Companies such as 3M, Caterpillar, Emerson, Ford, GM, Honeywell, Johnson Controls, Rockwell, Toro, and many others, have been in the industrial enterprise as their core business for several decades. Their alumni understand the problems and opportunities of the industrial enterprise unlike any others in any other region of the world. The hungry entrepreneurs studying machine learning, paired with vertical experts who have worked on these problems, comprise the ideal startup teams to build the IoT 3.0 applications. The Upper Midwest uniquely provides this talent.
- Adopters/enablers. While the industrial enterprise companies themselves may have limited appetites for leading innovation, they understand that market inflection is around the corner, and they are prepared to have their customers lead the way to achieving market alignment. Partnerships with these companies through co-investments, pilots, and sales affiliation to reach their customers and insert the innovations with minimal risk is the most effective path to adoption.
- Capital. Channels for entrepreneurial capital include venture funds, incubators and accelerators, and corporate investment funds. Of these, we believe that the first two provide the most efficient path for innovators, and that they create the on-ramp for in-house corporate teams to acquire well-formed companies that have demonstrated a strong product-market fit and, through later-stage funding, have even scaled their businesses. The Silicon Valley startups of yesterday that comprise some of the biggest market caps today have done exactly that. We believe that over an extended period, the Industrial Internet can deliver similar outcomes in the Upper Midwest.
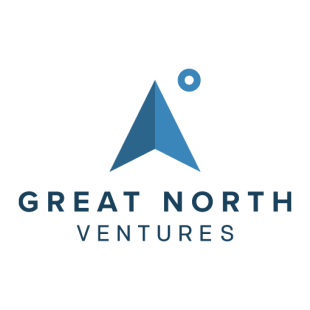
April Newsletter
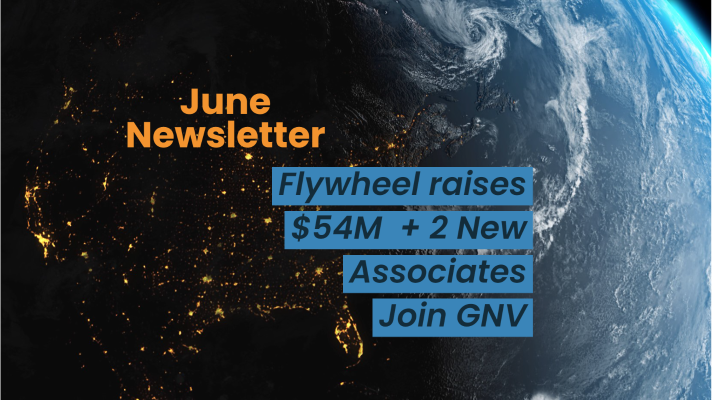
June Newsletter
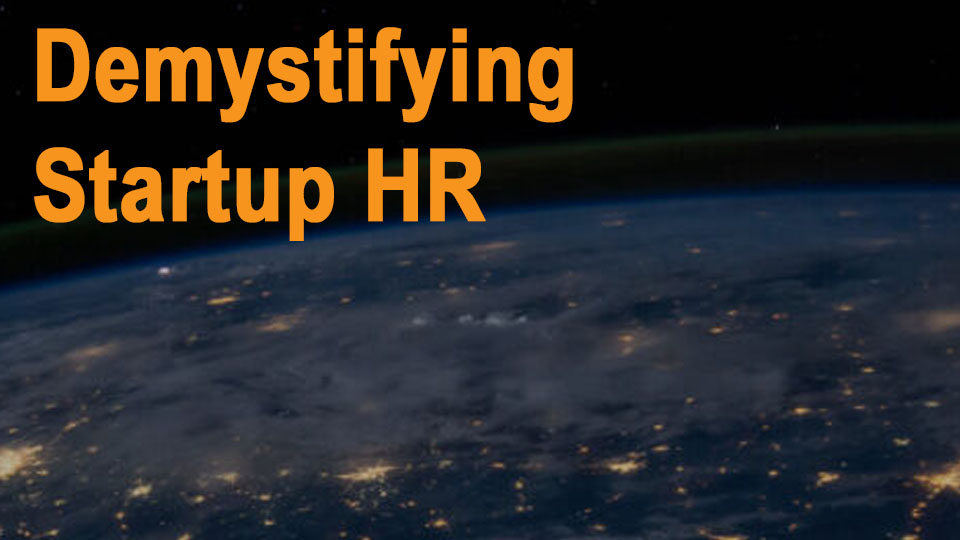
Demystifying Startup HR
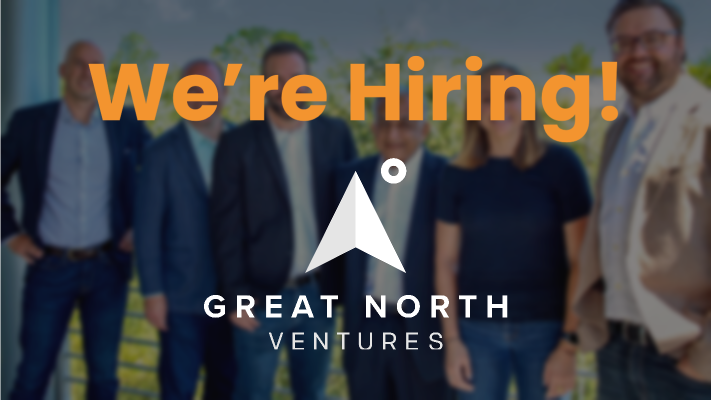
Head of Finance & Fund Administration- Venture Capital Firm (Remote)
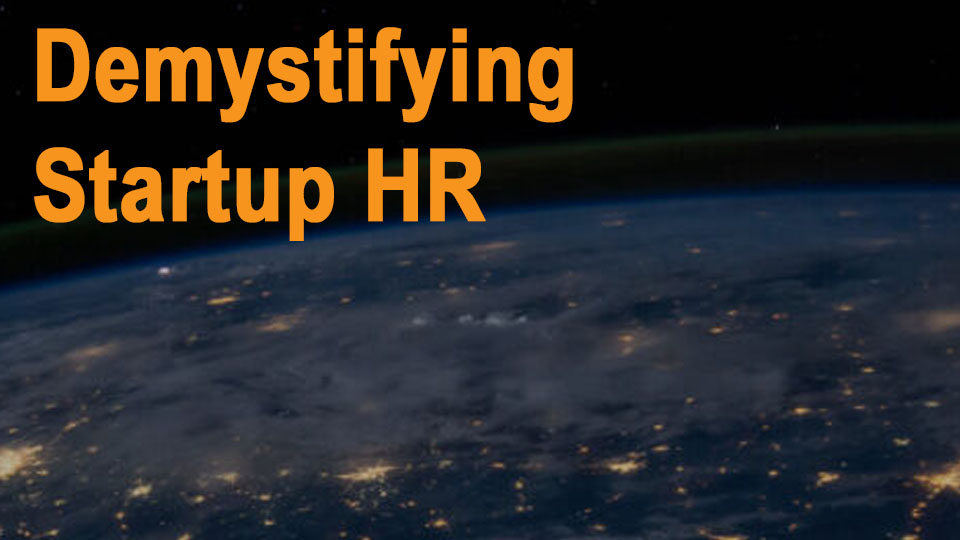
Demystifying Startup HR
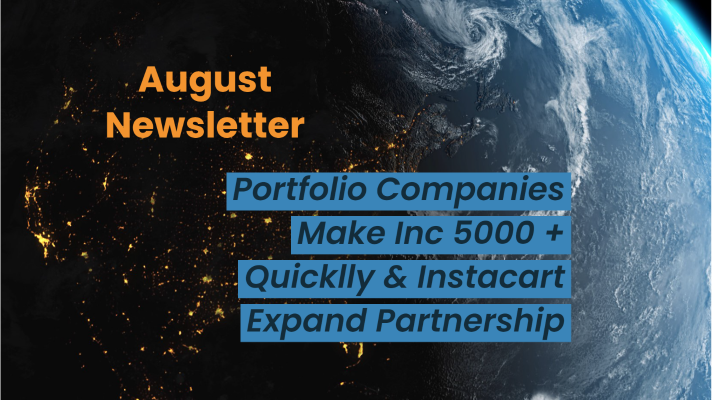
3 Portfolio Companies Make Inc. 5000 + Quicklly & Instacart Expand
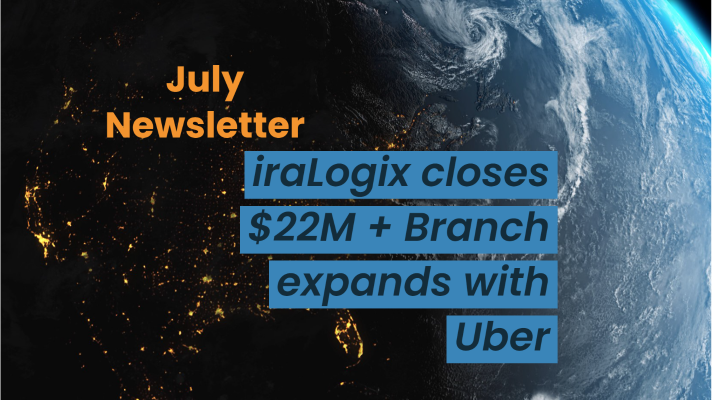
iraLogix closes $22M + Branch expands with Uber
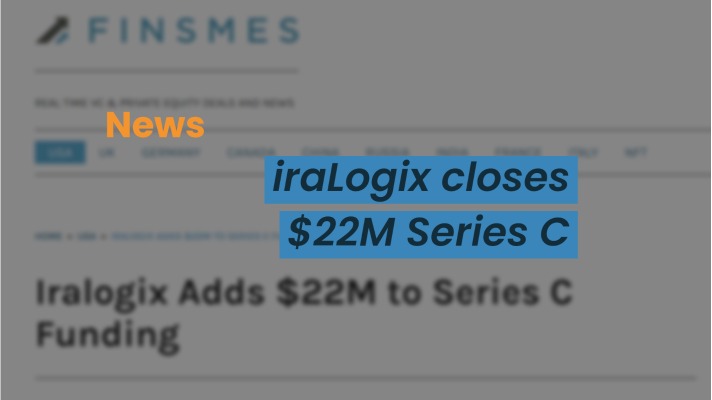
iraLogix closes $22M Series C
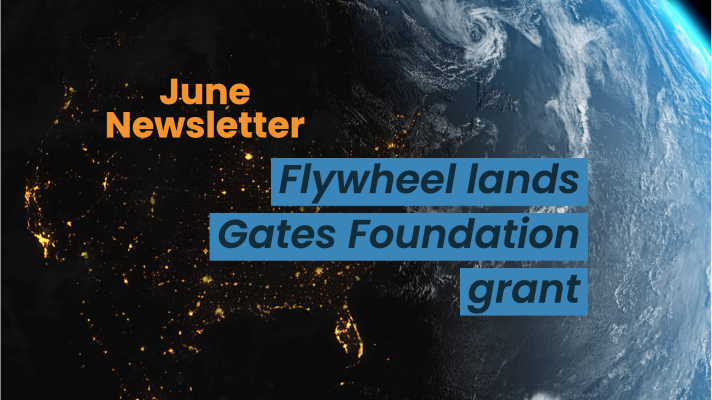
Flywheel lands Gates Foundation grant
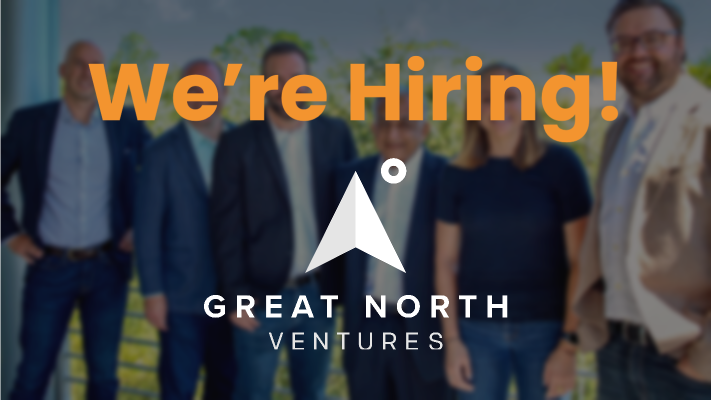
Venture Capital Analyst
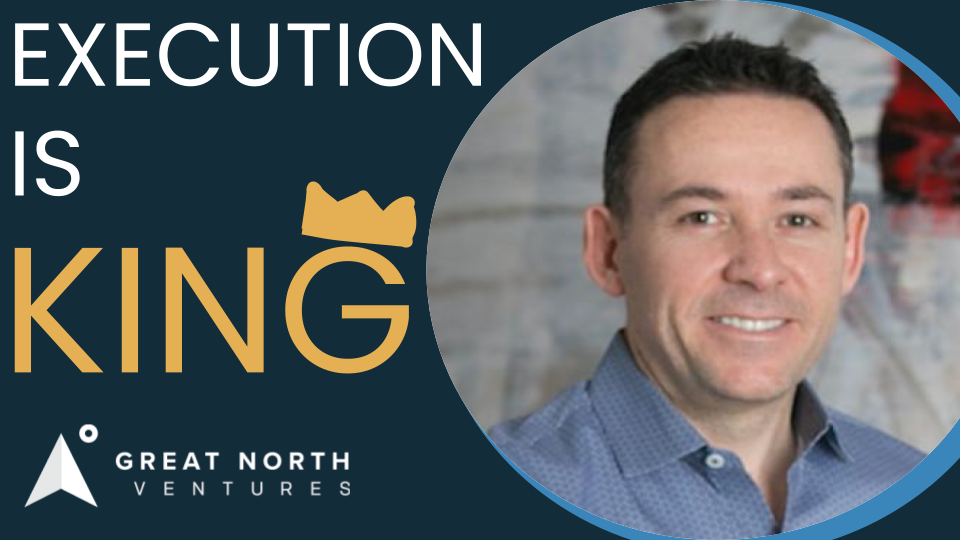
Orazio Buzza, Founder and CEO of Fooda – on Episode 13, “Execution is King”
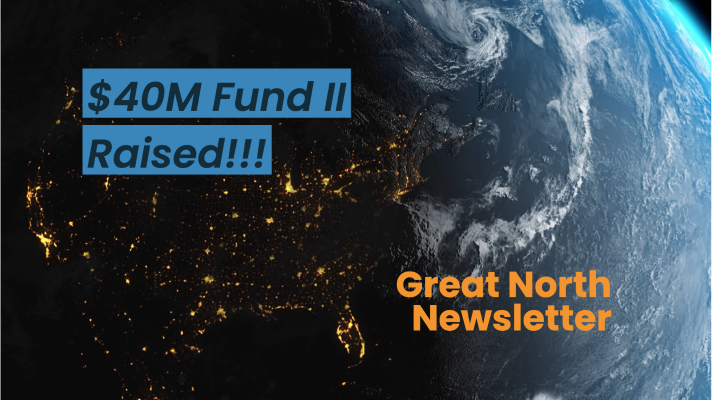
$40M Fund II Raised!
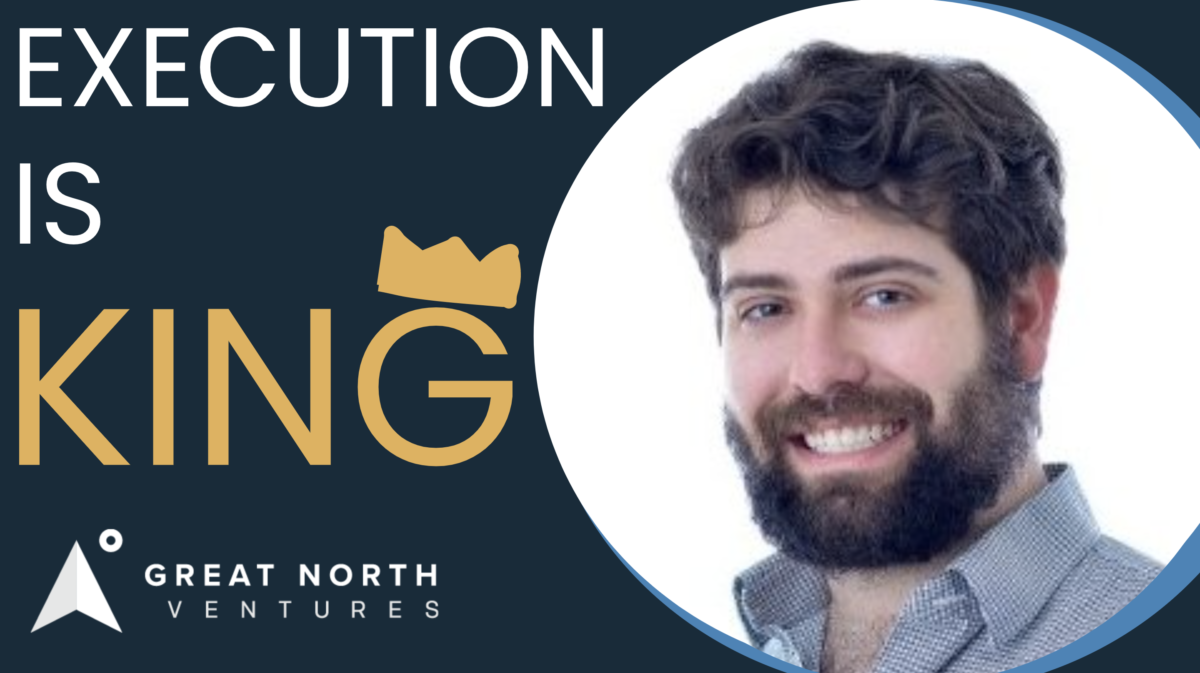
Eric Martell, Founder of Pear Commerce: Episode 13, Execution is King
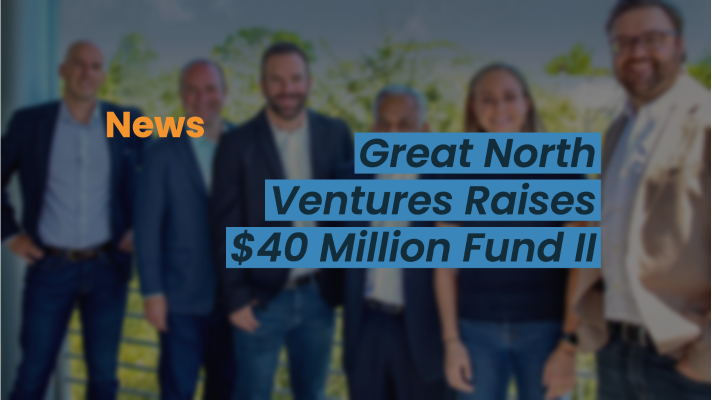
Great North Ventures Raises $40 Million Fund II
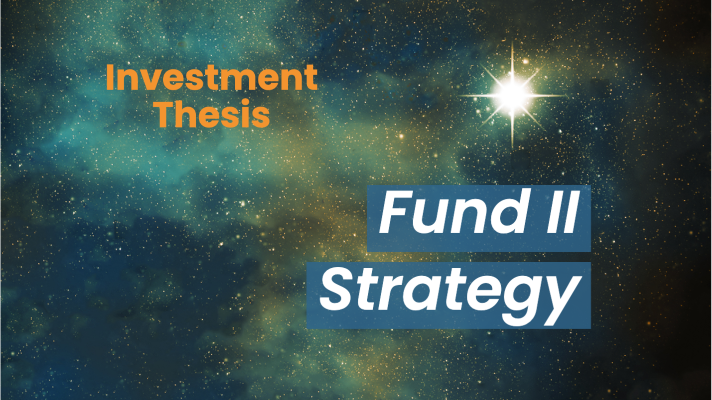
Investment Thesis: Fund II Strategy
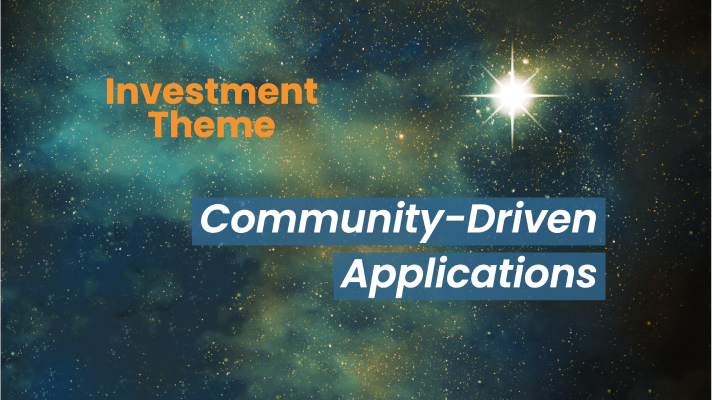
Investment Theme: Community-Driven Applications
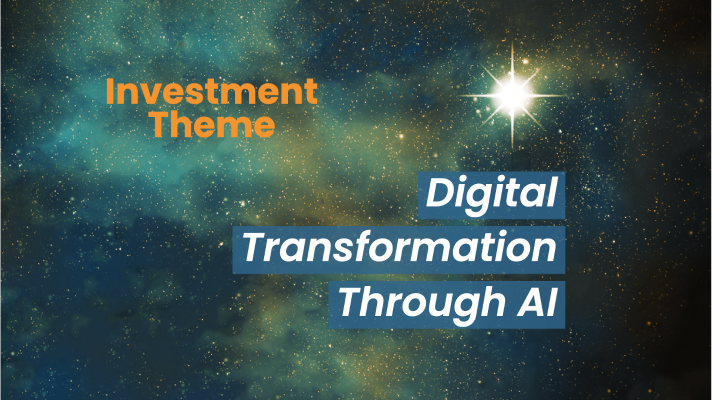
Investment Theme: Digital Transformation Through AI
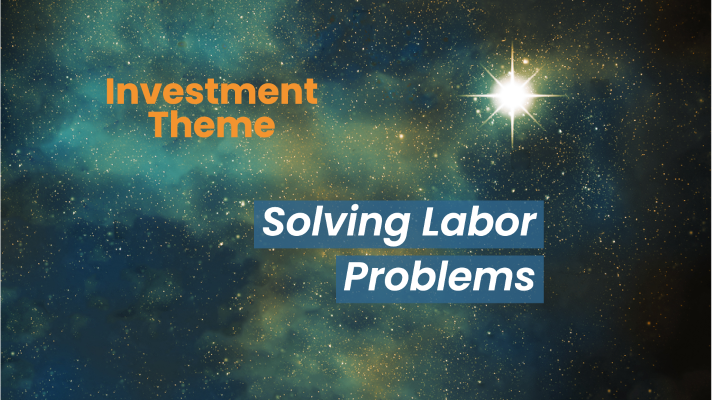
Investment Theme: Solving Labor Problems
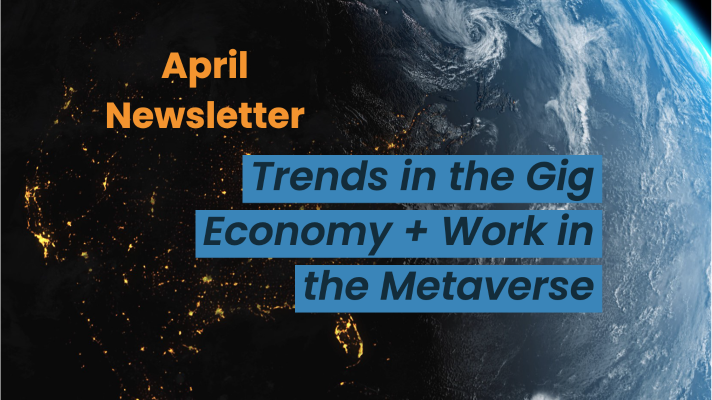
Trends in the Gig Economy + Work in the Metaverse
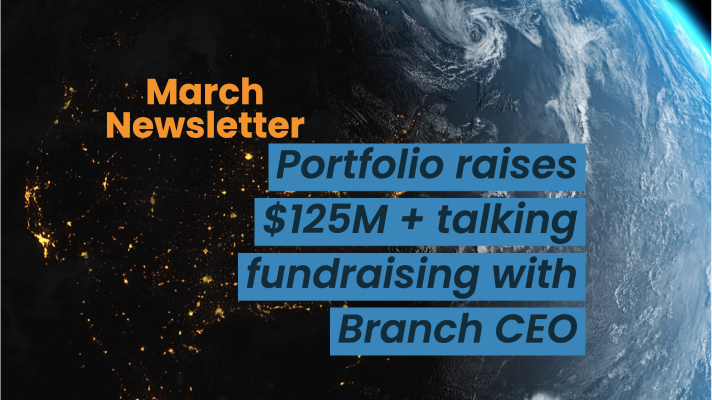
Portfolio raises $125M + talking fundraising with Branch CEO
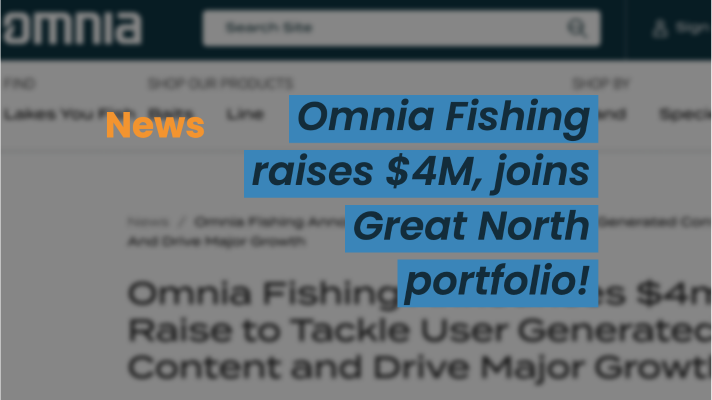
Omnia Fishing closes $4M round, joins Great North portfolio!
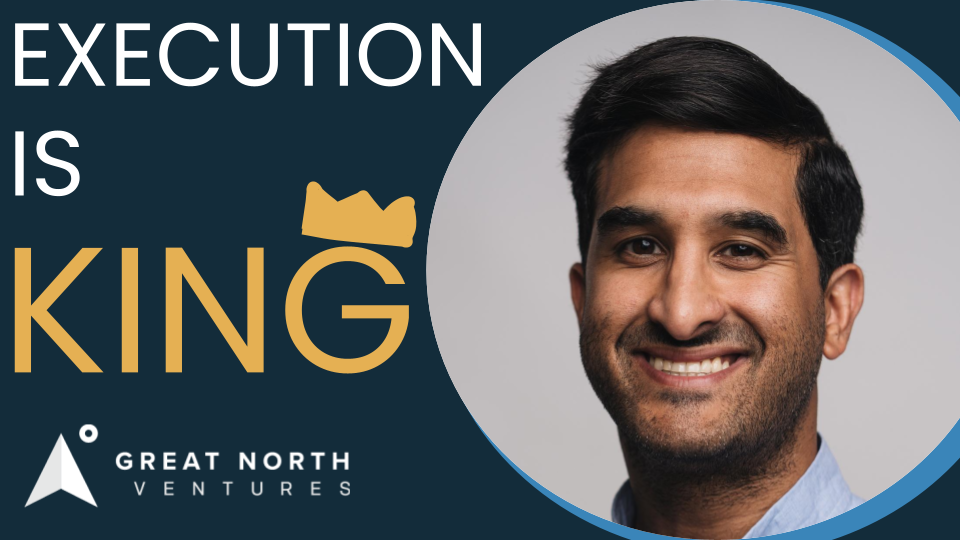
Atif Siddiqi, Founder/CEO of Branch: Episode 11, Execution is King
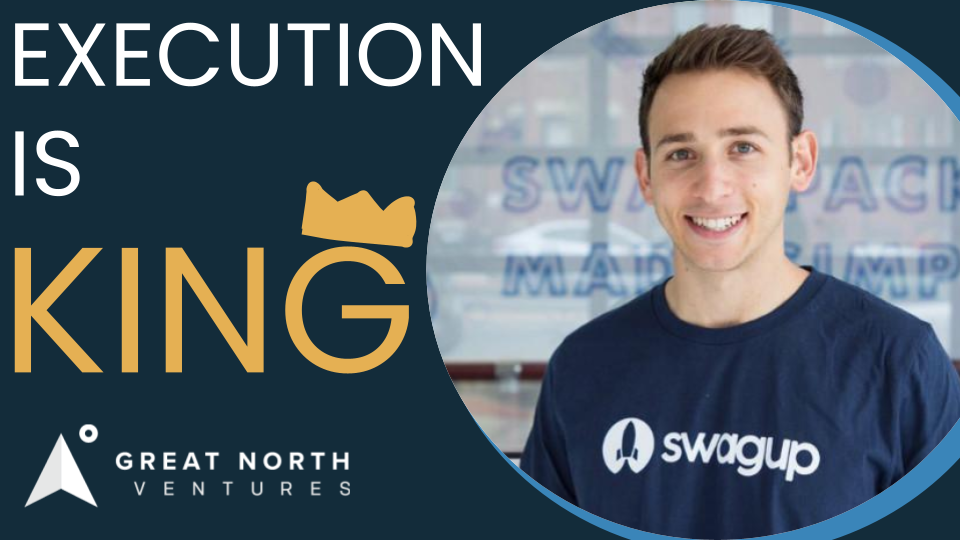
Michael Martocci, CEO and Founder of SwagUp: Episode 10, Execution is King
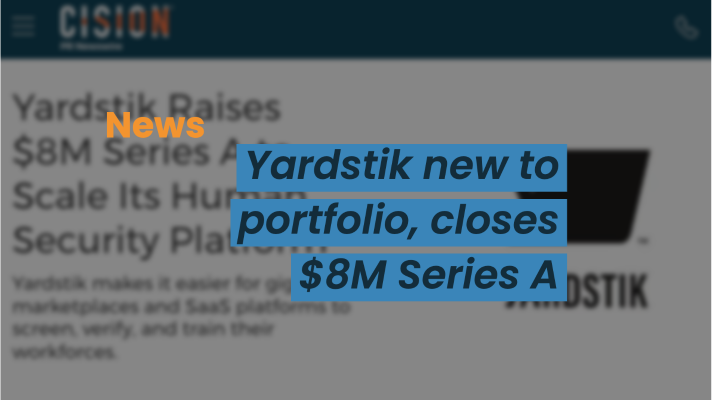
Yardstik new to portfolio, closes $8M Series A
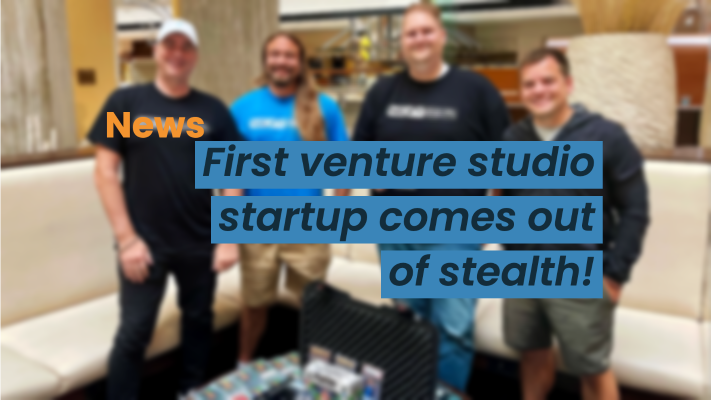
First venture studio startup comes out of stealth!
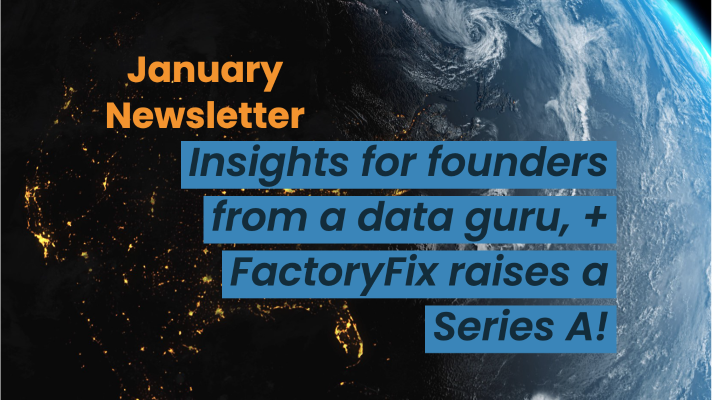
Insights for founders from a data guru, + FactoryFix raises a Series A!
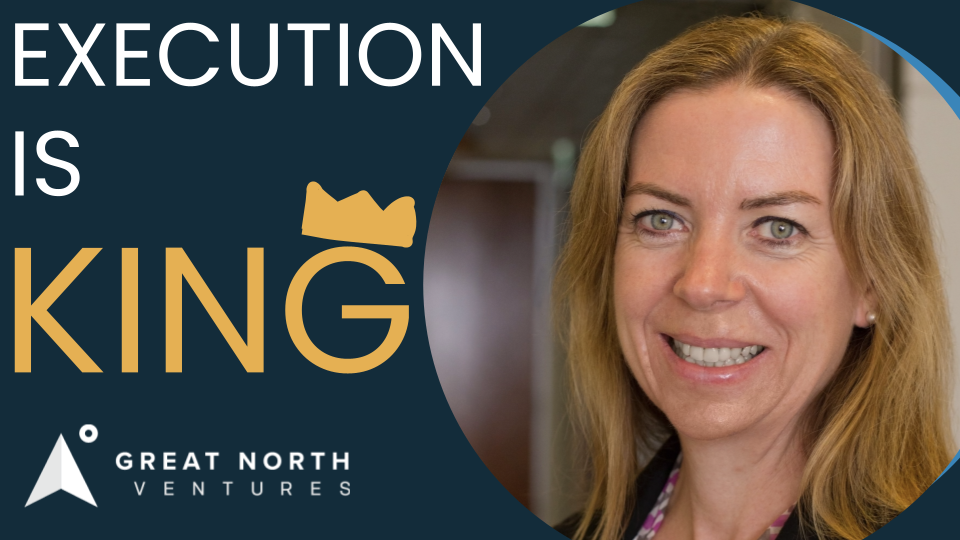
Una Fox: Episode 9, Execution is King
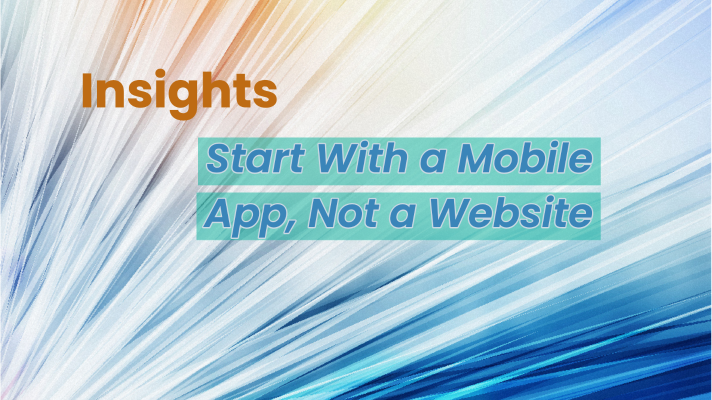
Start With a Mobile App, Not a Website
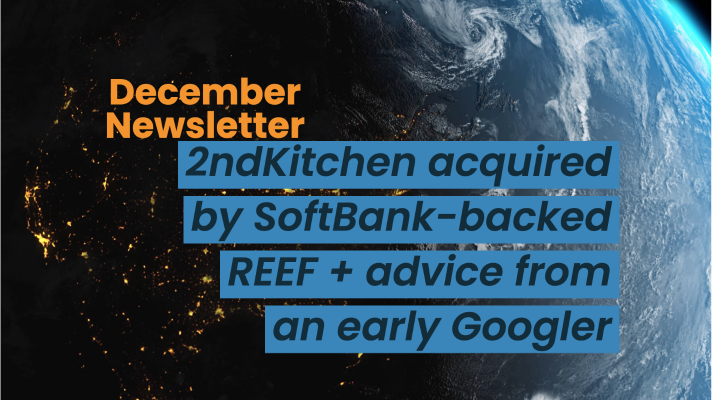
2ndKitchen acquired by SoftBank-backed REEF + advice from an early Googler
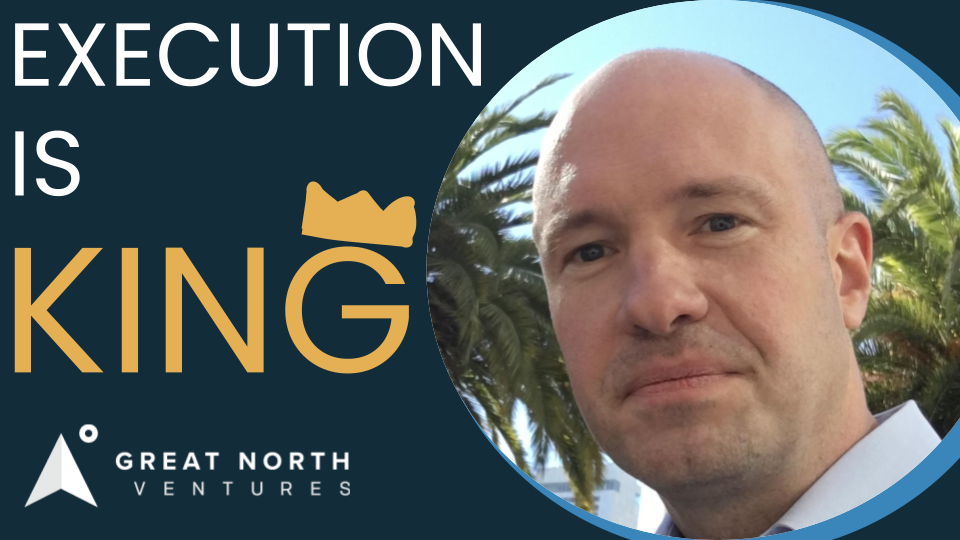
Joe Sriver, 4giving: Episode 8, Execution is King
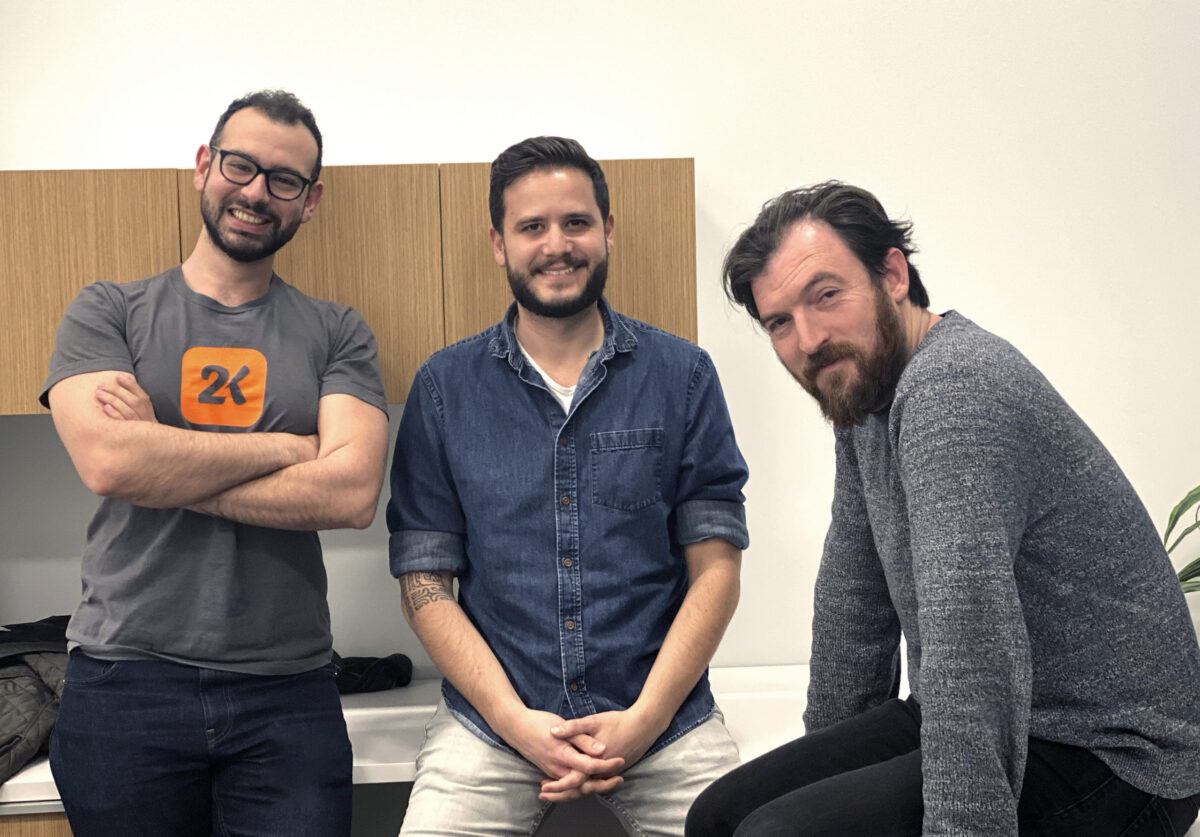
2ndKitchen Acquired by REEF
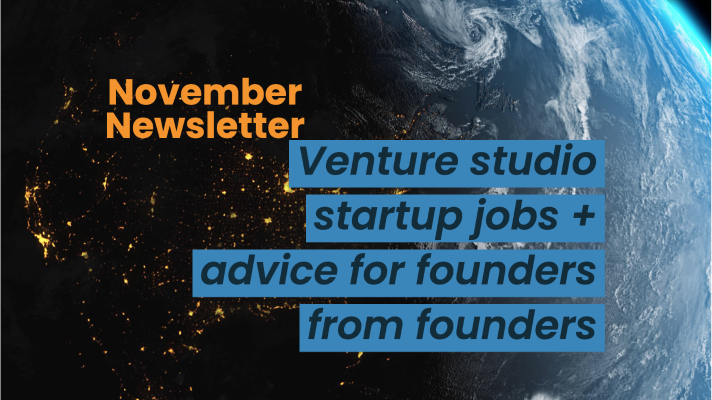
Venture studio startup jobs + advice for founders from founders
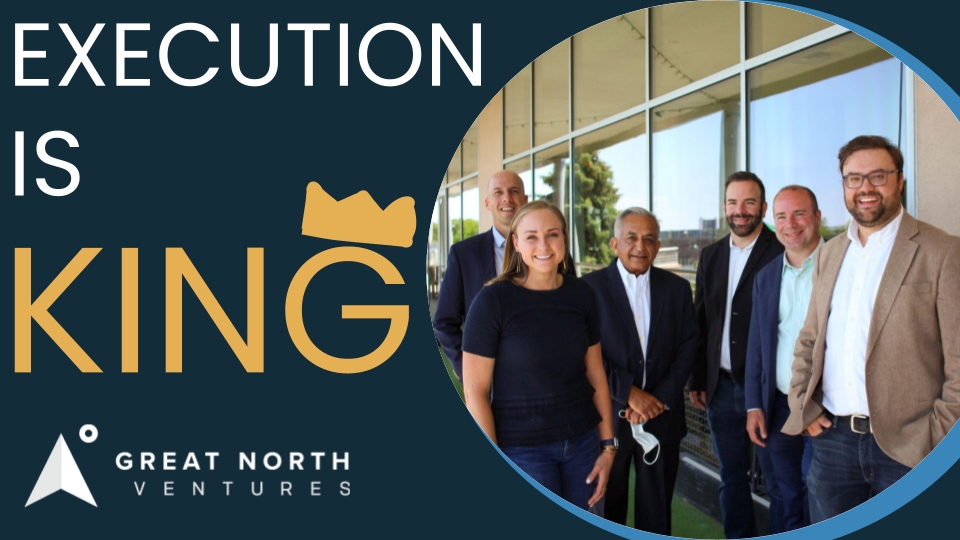
Best Advice from the Great North Annual Event: Episode 7, Execution is King
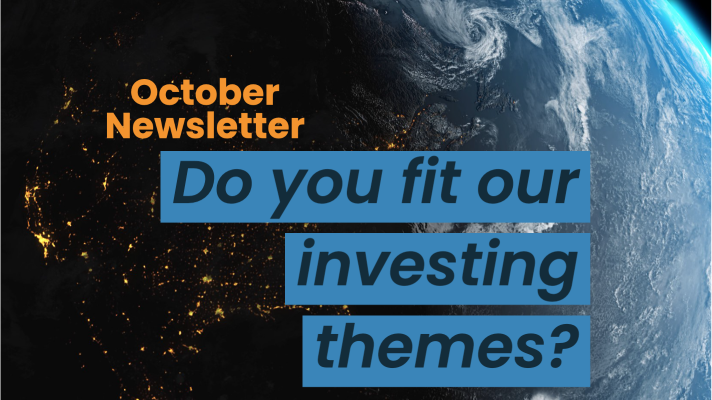
Newsletter: Do you fit our investing themes?
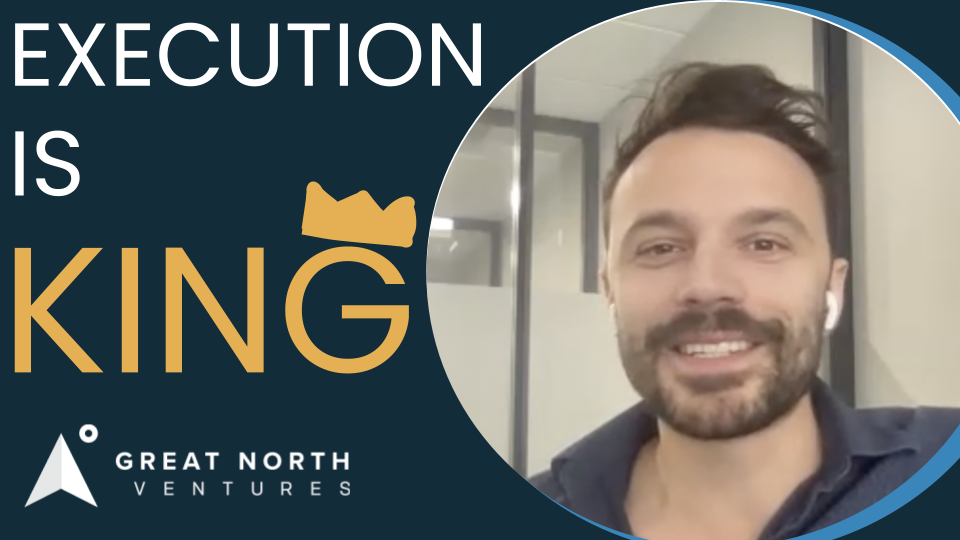
Jonathan Treble, PrintWithMe: Episode 6, Execution is King
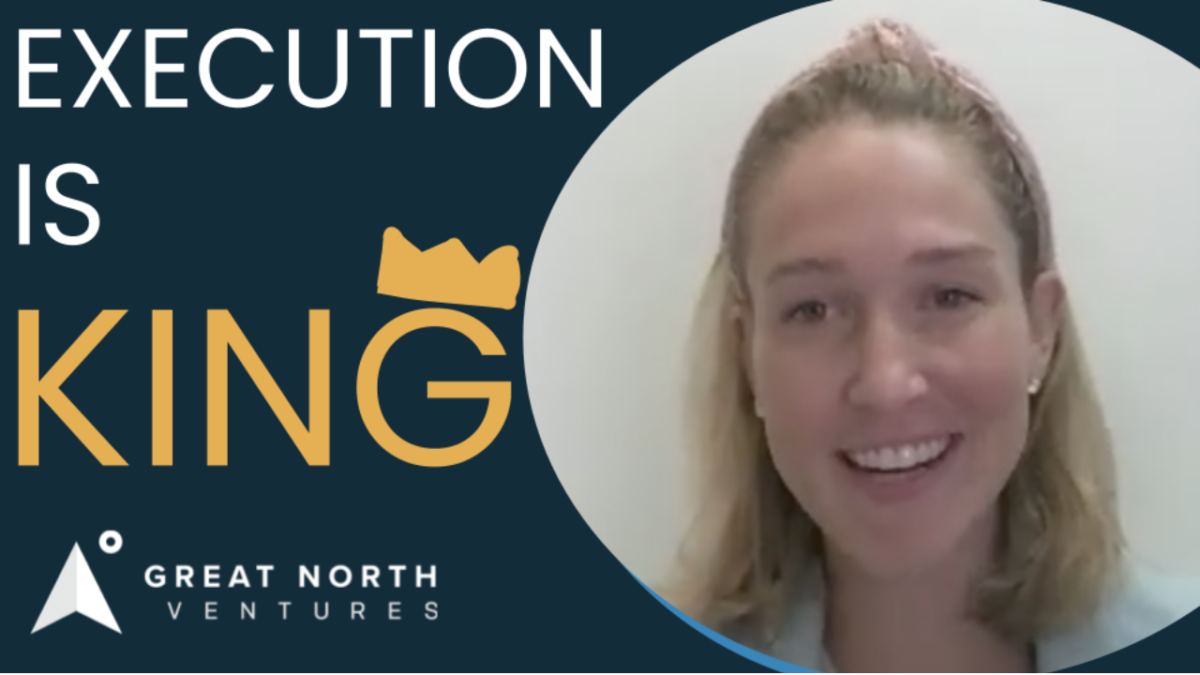
Anna Mason, Revolution: Episode 5, Execution is King
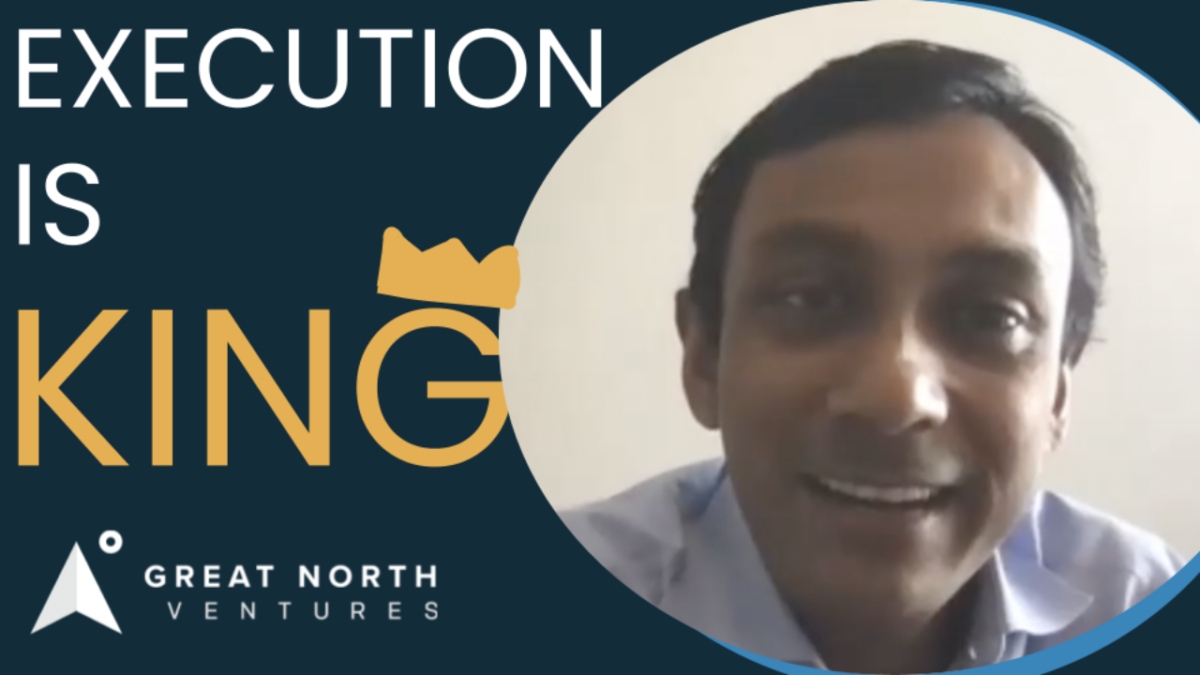
Mynul Khan, FieldNation: Episode 4, Execution is King
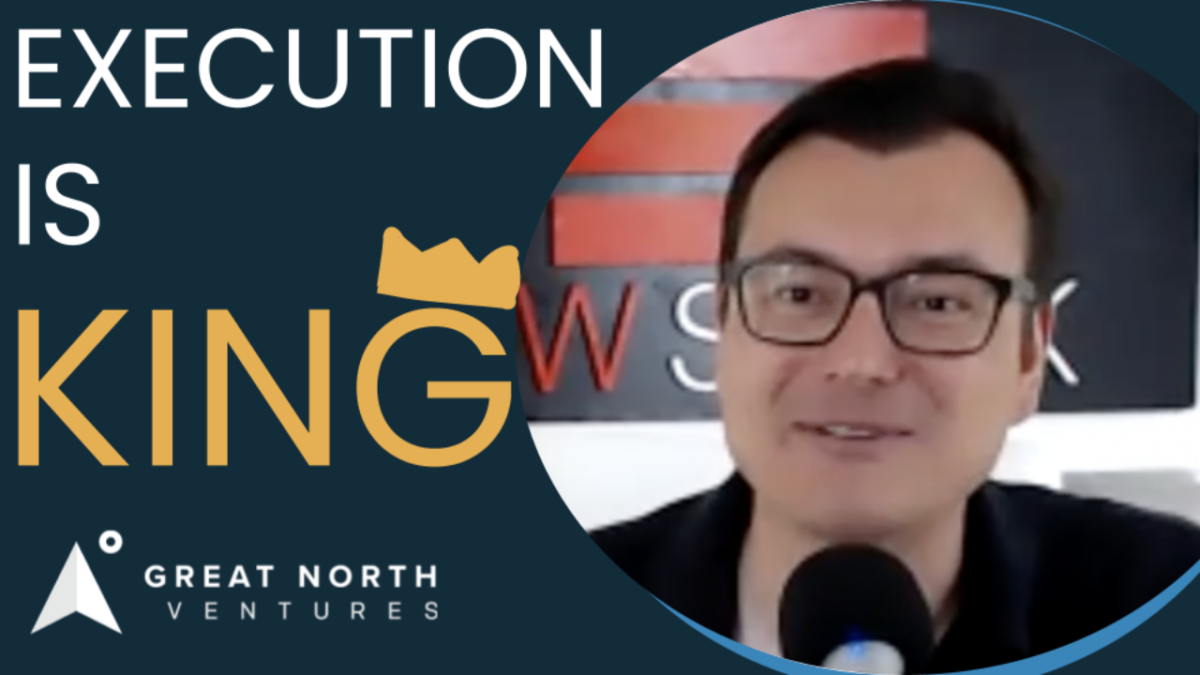
Nick Moran, New Stack Ventures: Episode 3, Execution is King
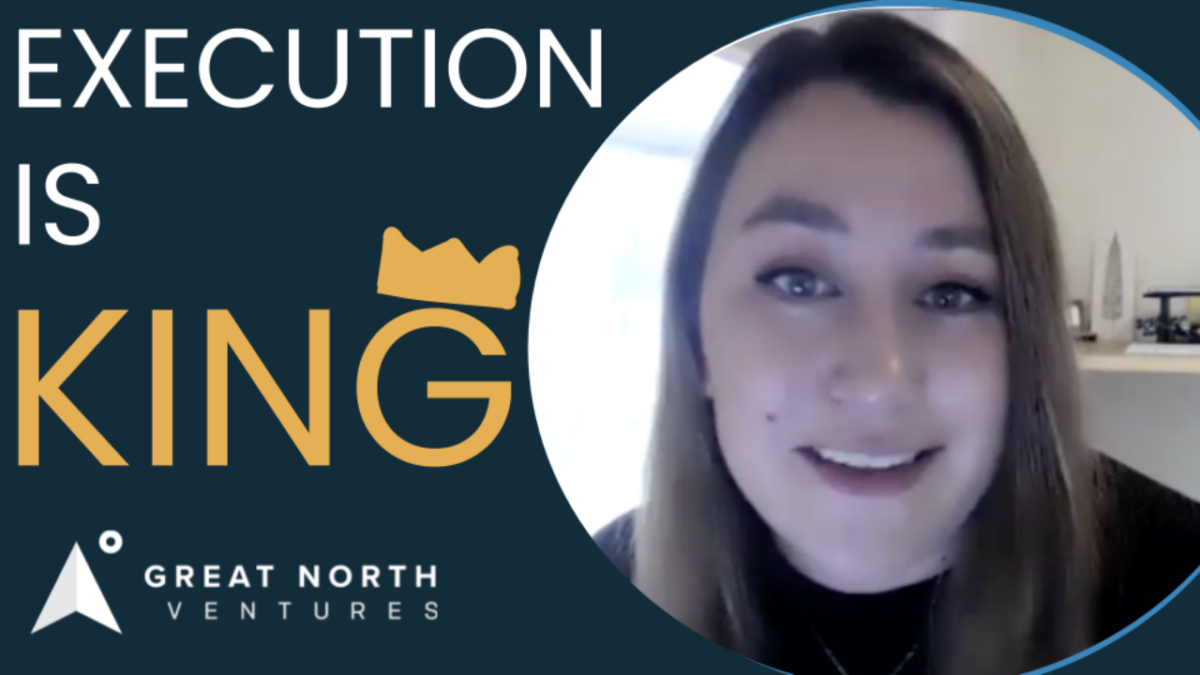
Molly Pyle, Center on Rural Innovation (CORI): Episode 2, Execution is King
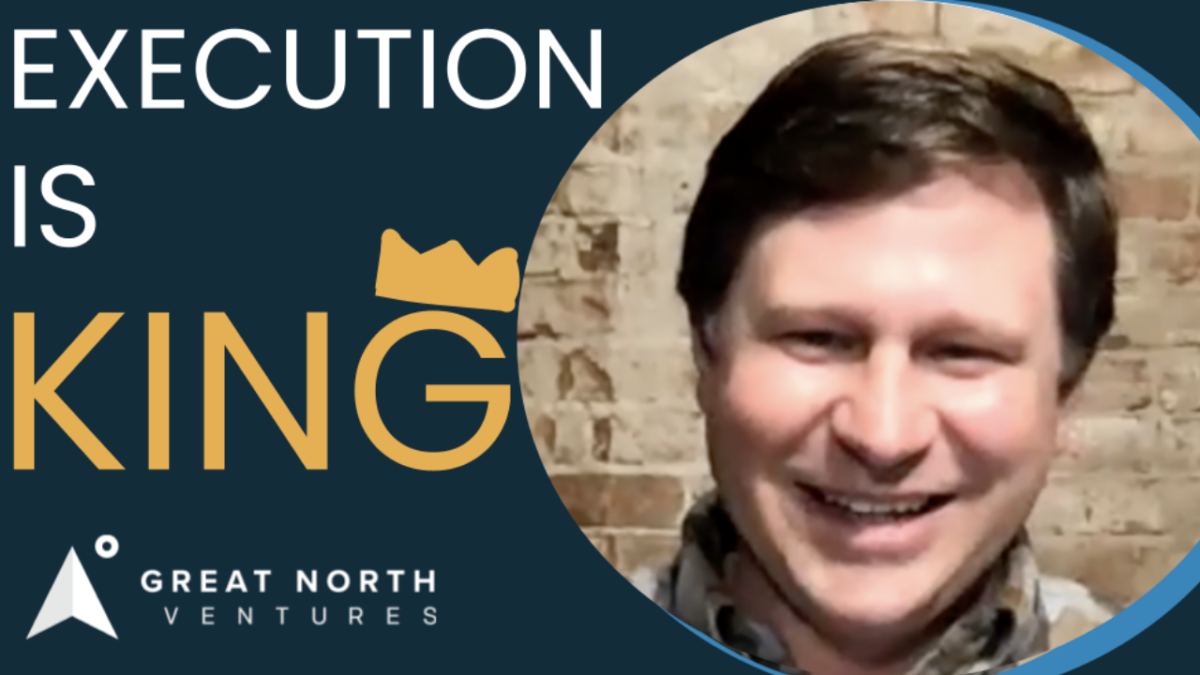
Justin Kaufenberg, Rally Ventures: Execution is King Episode 1
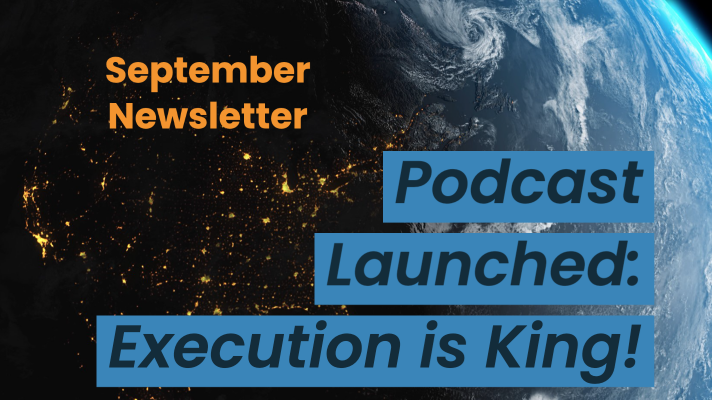
Newsletter: “Podcast Launched: Execution is King!”
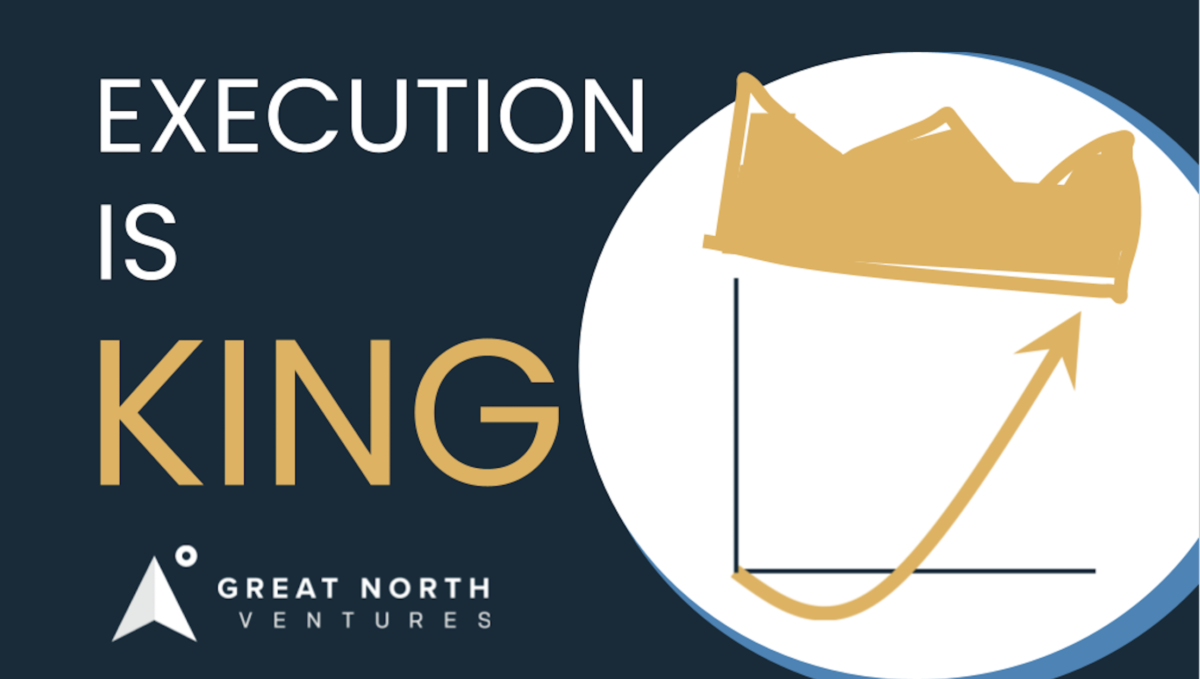
“Execution is King” – the Great North Ventures Podcast
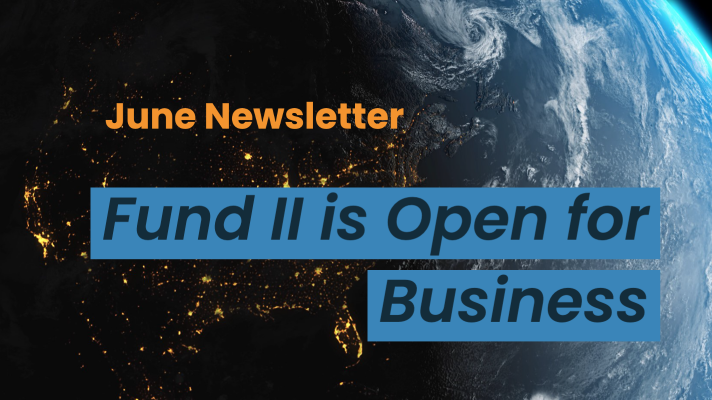
Newsletter: Fund II is open for business!
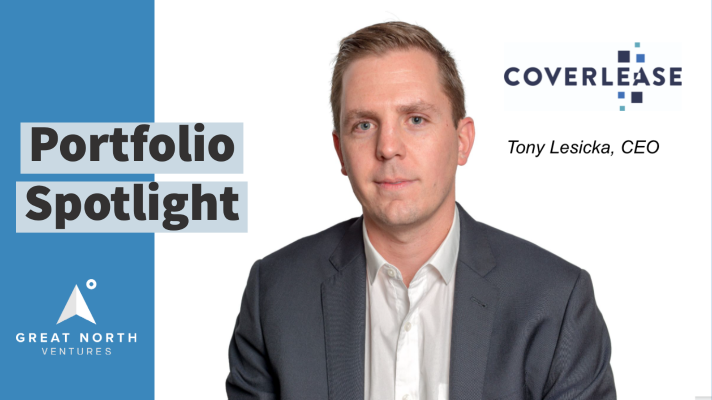
Unlocking the Potential of Anonymized Commercial Real Estate (CRE) Data
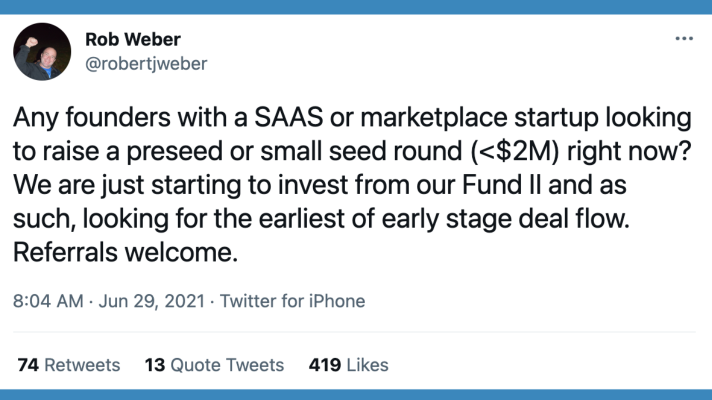
Fund II is open for Business!
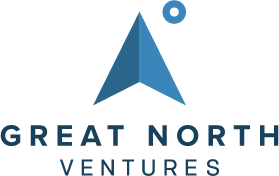
Mike Schulte Promoted to Venture Partner
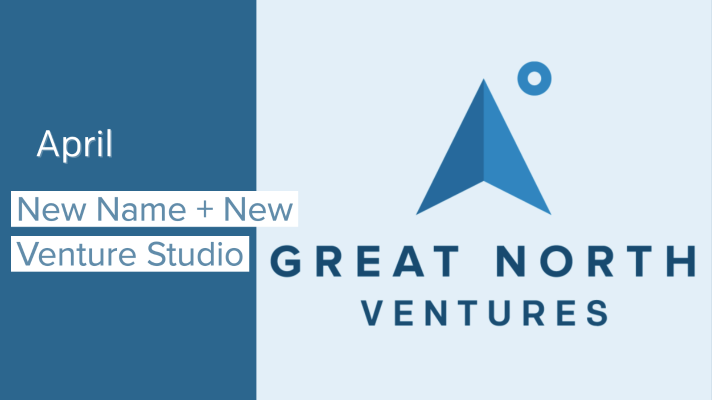
New Name + New Venture Studio
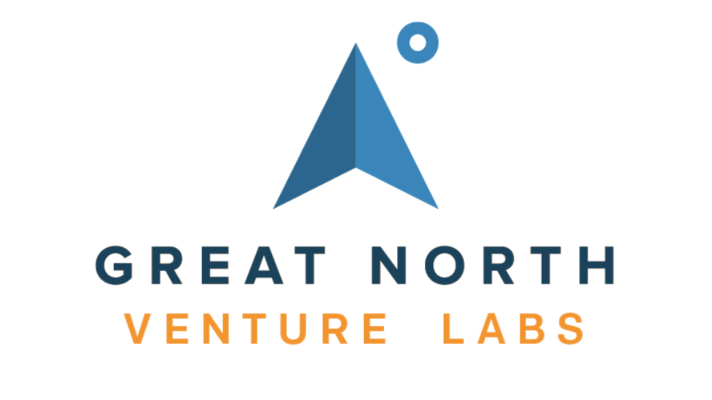
Great North Launches Startup Studio
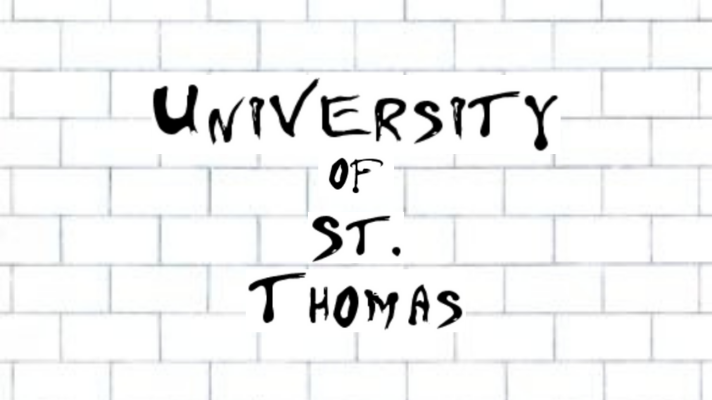
We Don’t Need No [full-time MBA] Education
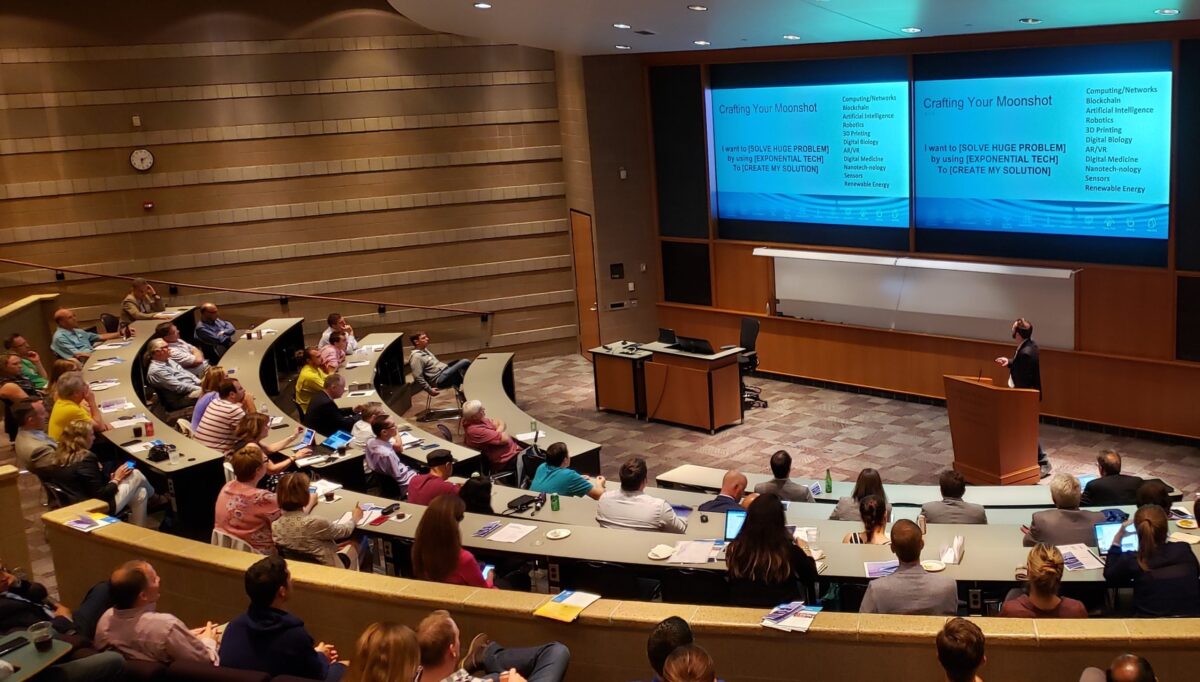
How the University of Minnesota is Embracing Startup Culture
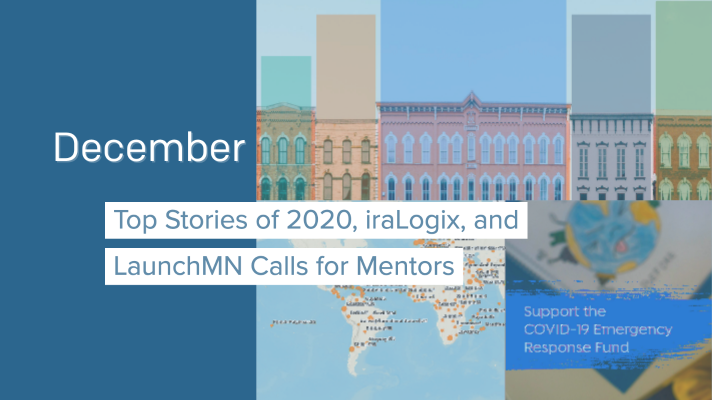
Top Stories of 2020, iraLogix, and LaunchMN Calls for Mentors
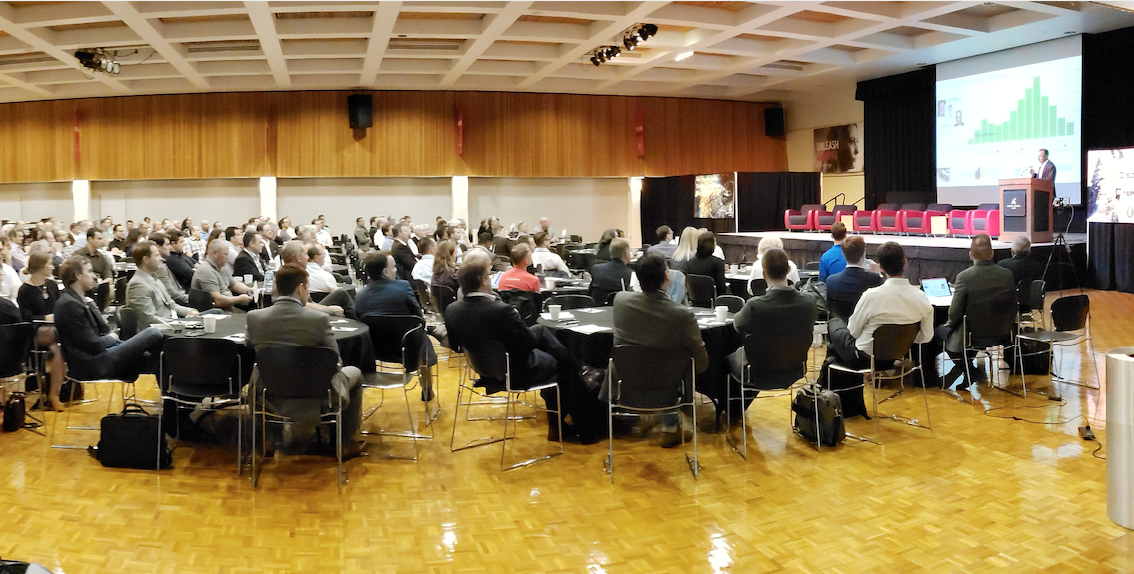
Building Capacity for Innovation
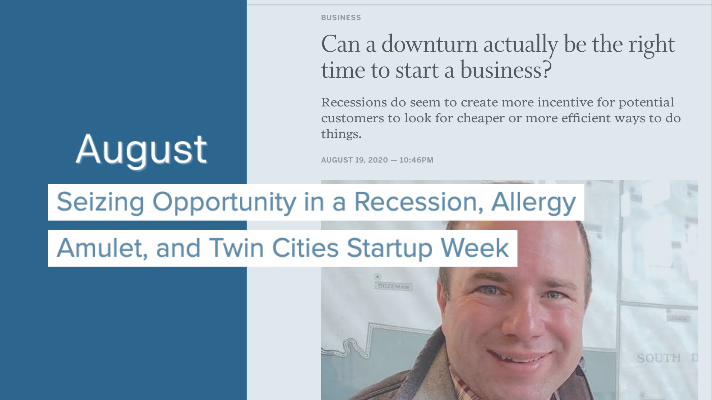
Seizing Opportunity in a Recession, Allergy Amulet, and Twin Cities Startup Week
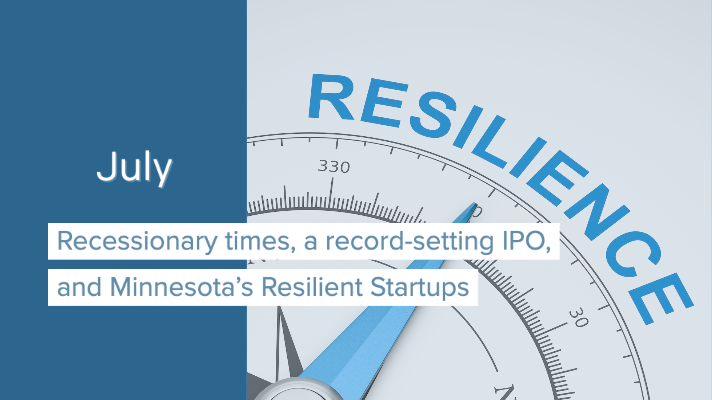
Recessionary times, a record-setting IPO, and Minnesota’s Resilient Startups
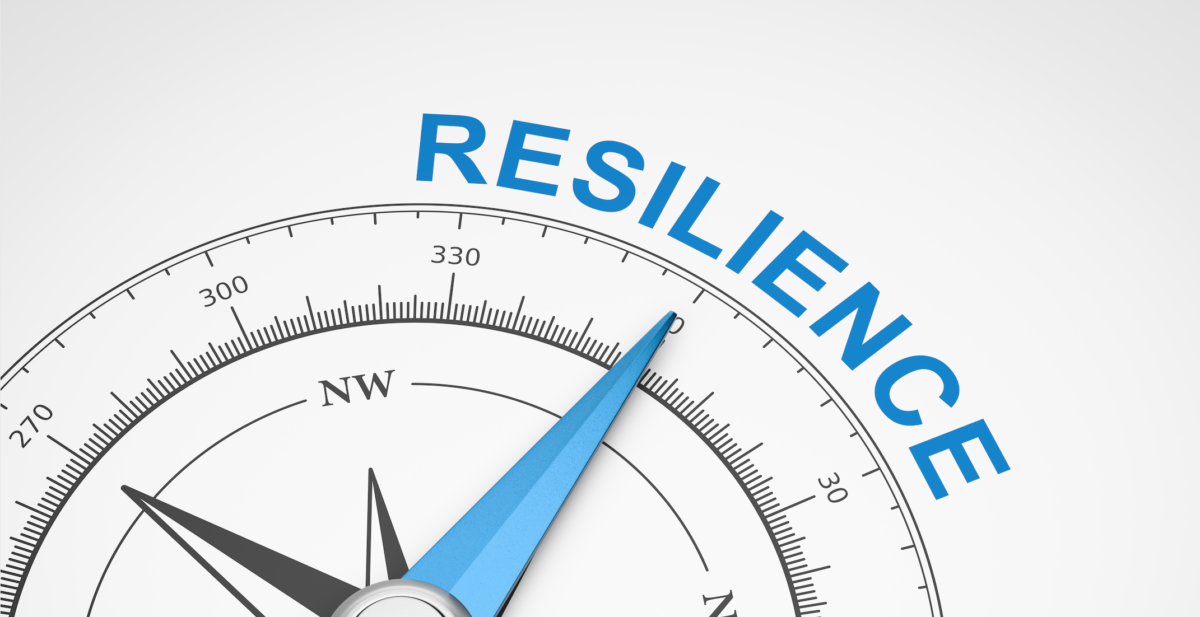
Minnesota's Resilient Startups
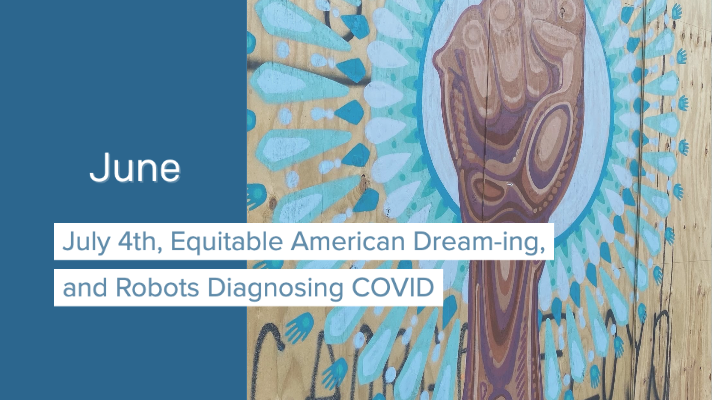
July 4th, Equitable American Dream-ing, and Robots Diagnosing COVID
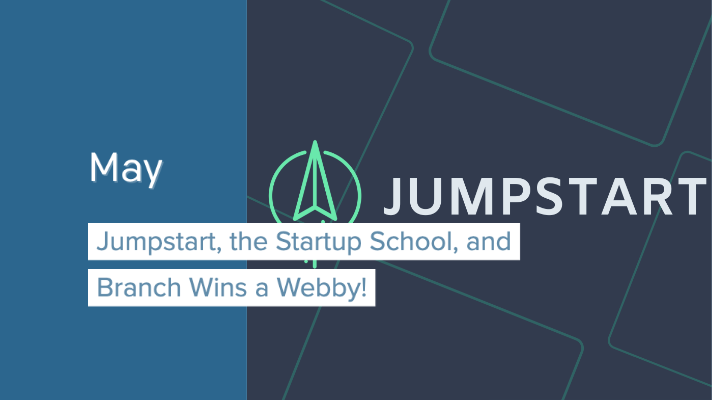
Jumpstart, the Startup School, and Branch Wins a Webby!
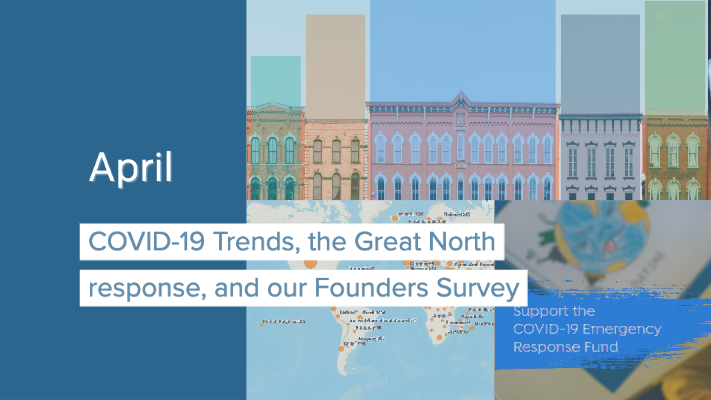
COVID-19 Trends, the Great North response, and our Founders Survey
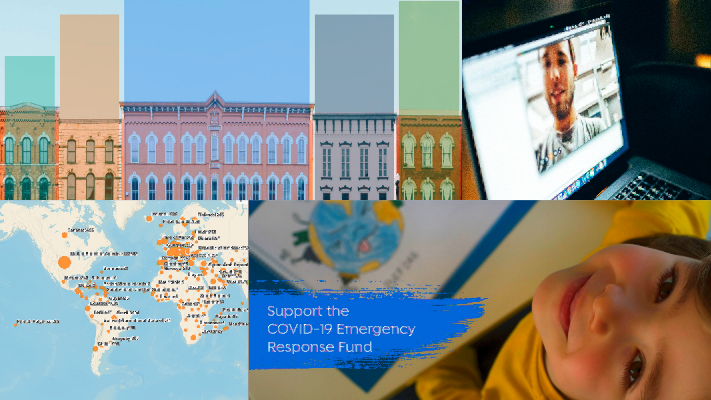
Giving in the Time of Coronavirus
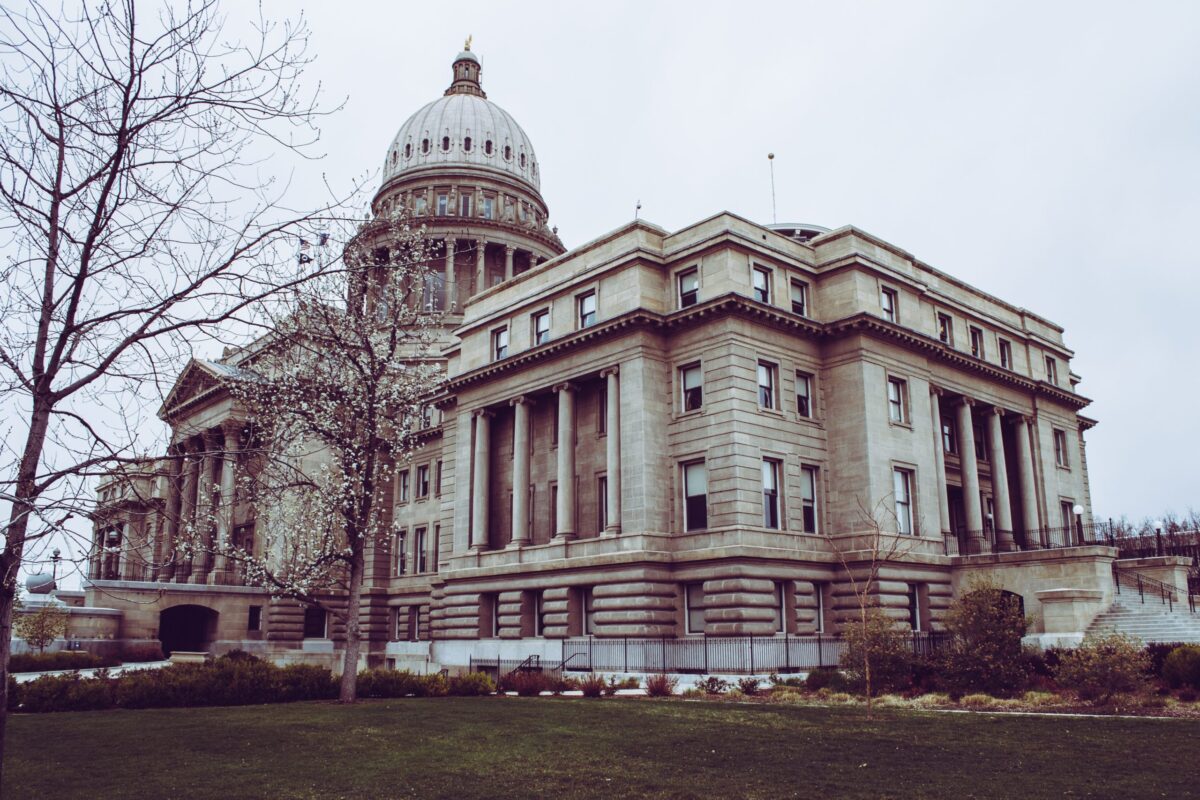
COVID-19 Resources for Startups, State-by-State
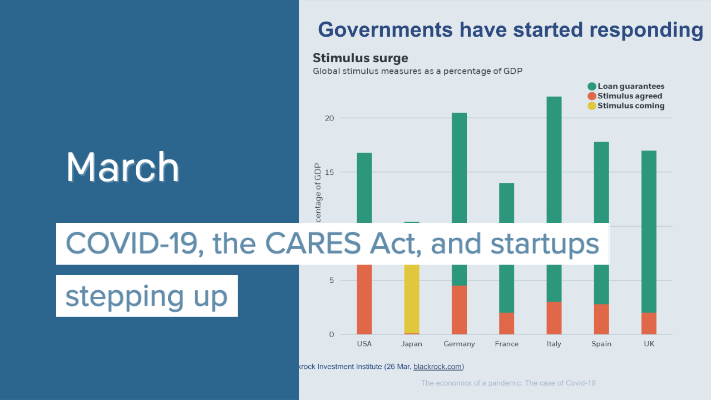
COVID-19, the CARES Act, and startups stepping up
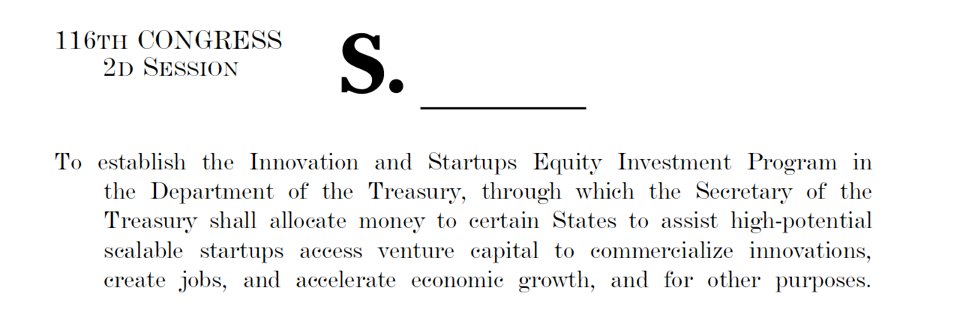
New Business Preservation Act
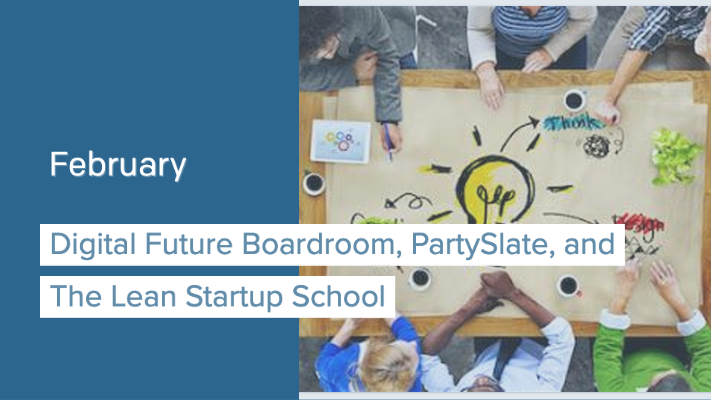
Digital Future Boardroom, PartySlate, and The Lean Startup School
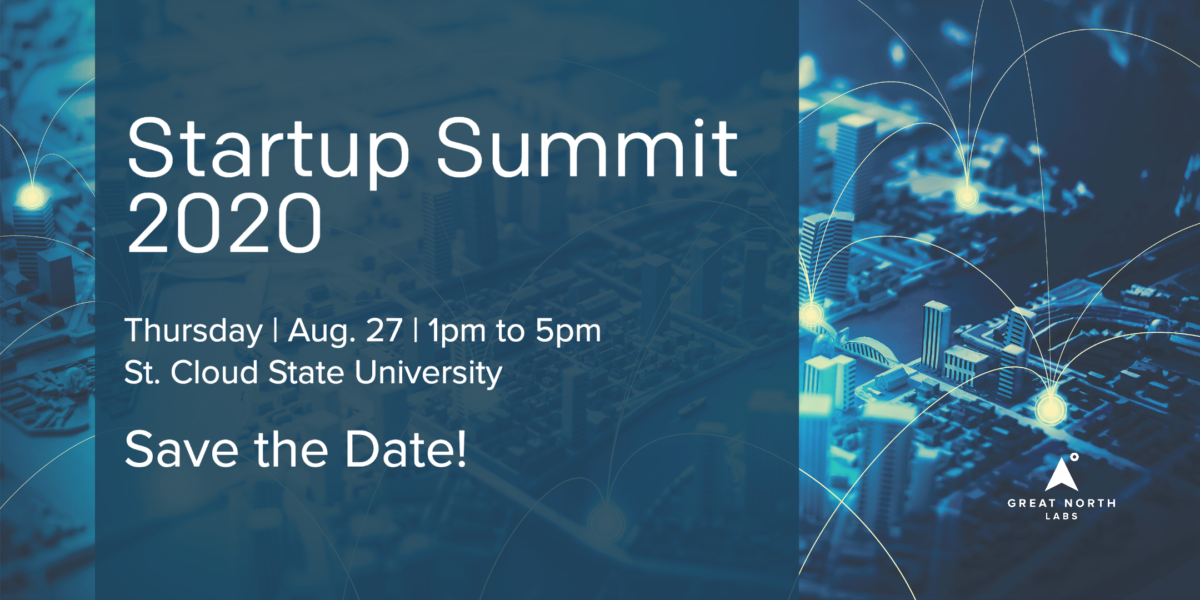
Great North Labs’s Startup Summit 2020
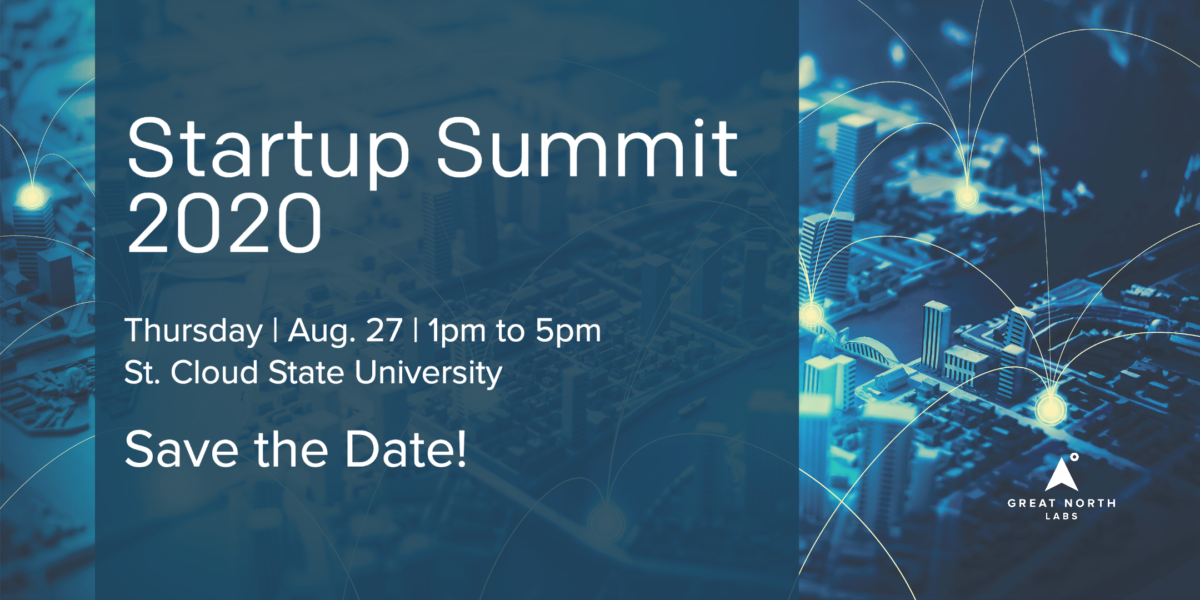
Great North Labs's Startup Summit 2020
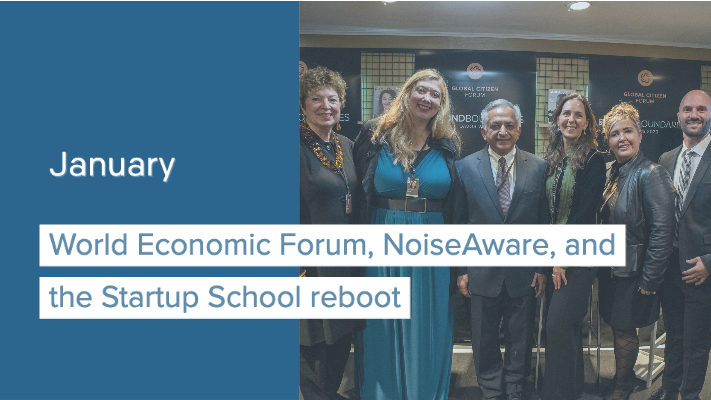
World Economic Forum, NoiseAware, and the Startup School reboot
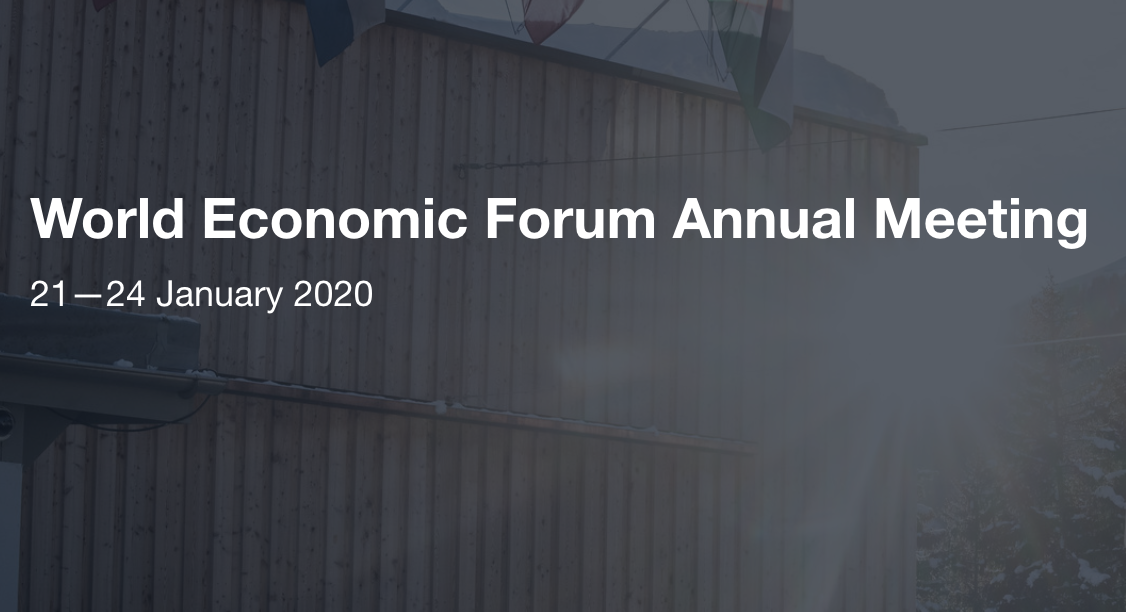
Great North Labs at the World Economic Forum 2020 in Davos
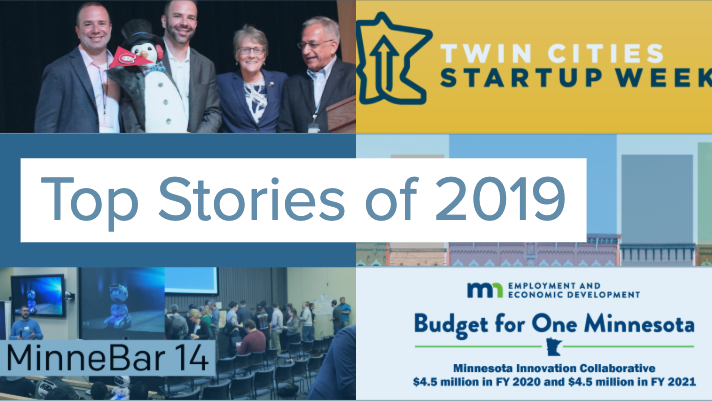
Top 5 Stories of 2019
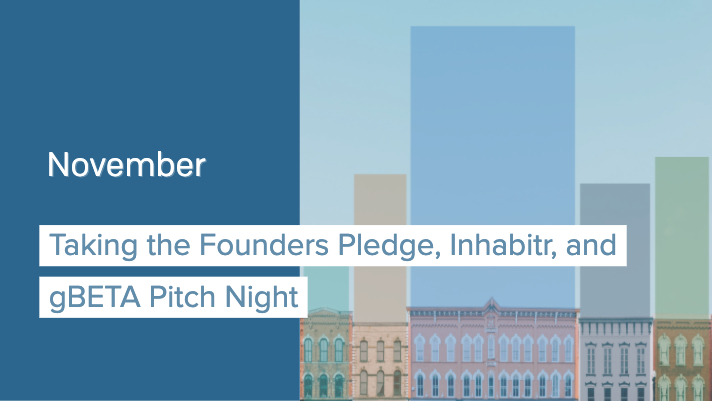
Taking the Founders Pledge, Inhabitr, and gBETA Pitch Night
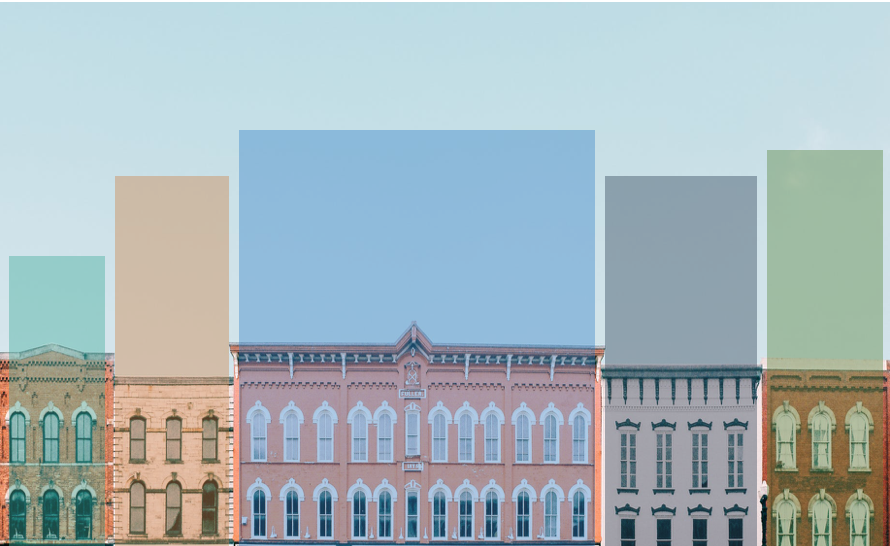
Founders Pledge: Support the Organizations that Support You
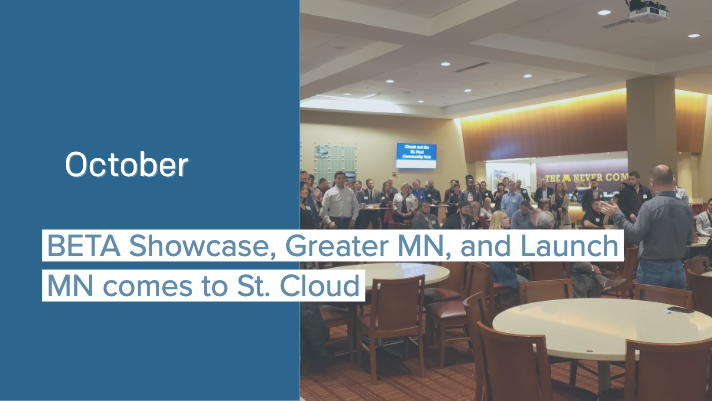
BETA Showcase, Greater MN, and Launch MN comes to St. Cloud
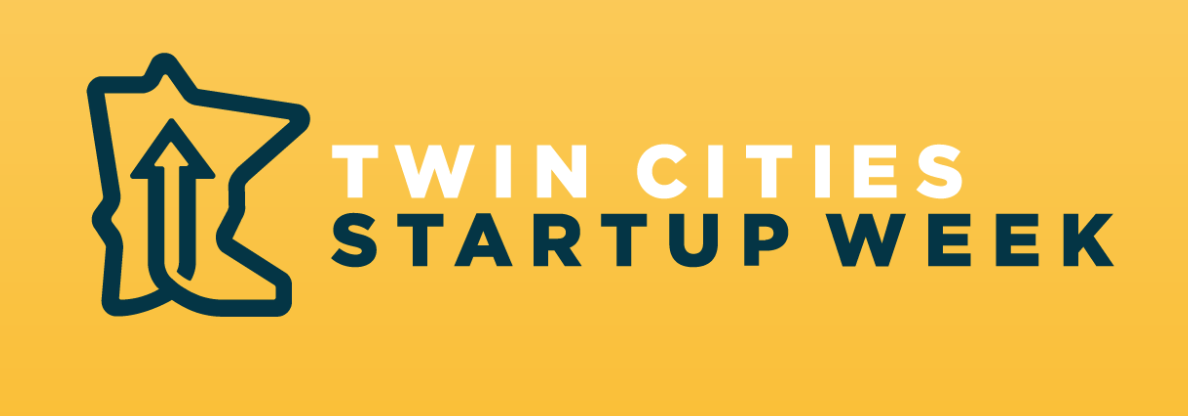
7 Places to Spot Us at Startup Week
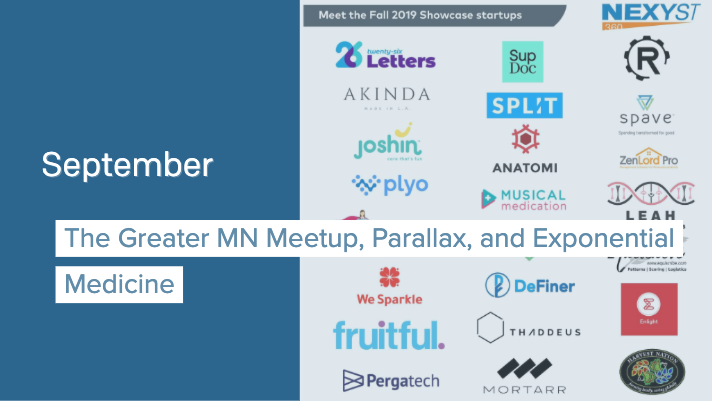
The Greater MN Meetup, Parallax, and Exponential Medicine
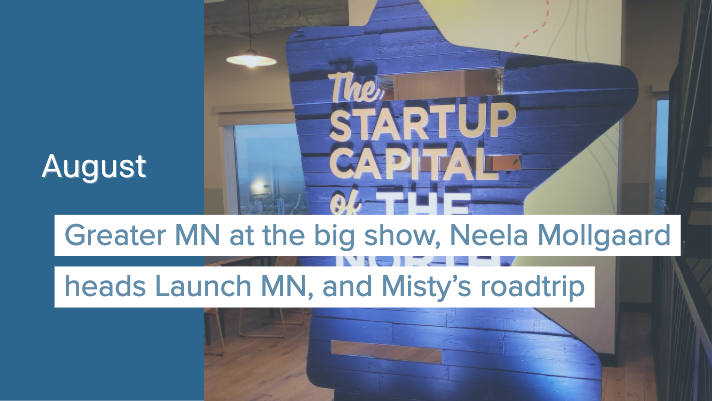
Greater MN at the big show, Neela Mollgaard heads Launch MN, and Misty’s roadtrip.
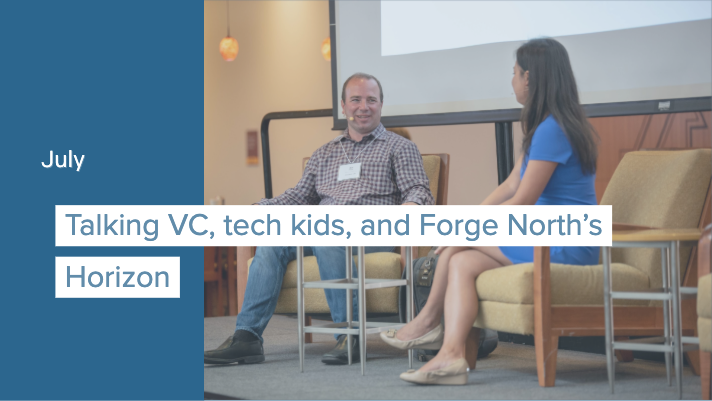
Talking VC, tech kids, and Forge North’s Horizon
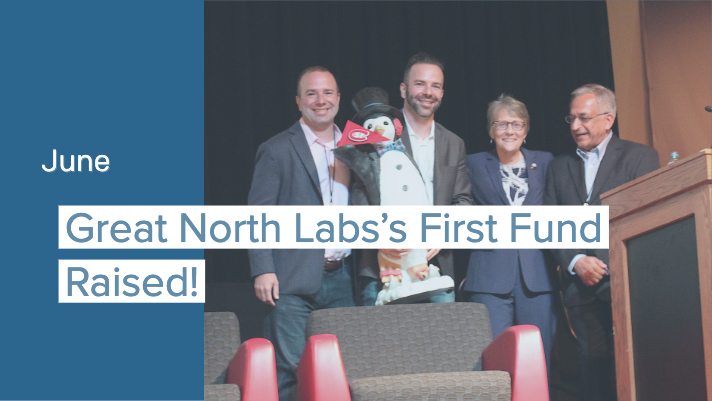
June: Great North Labs’s first fund raised!
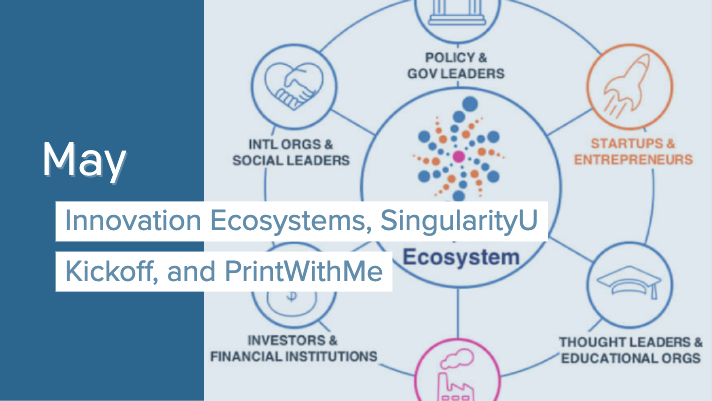
May: Innovation Ecosystems, SingularityU Kickoff, and PrintWithMe
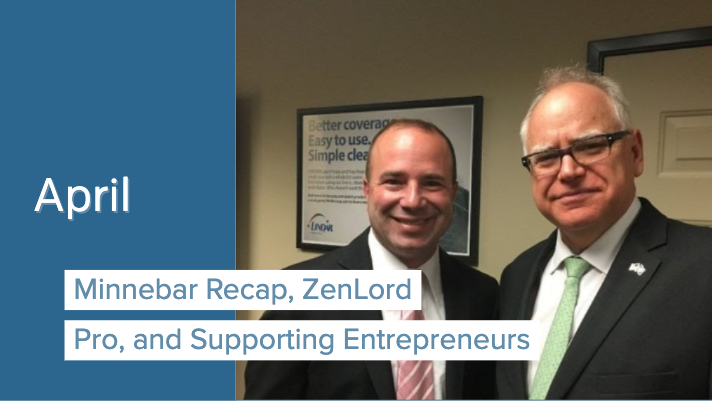
April: Minnebar Recap, ZenLord Pro, and Supporting Entrepreneurs
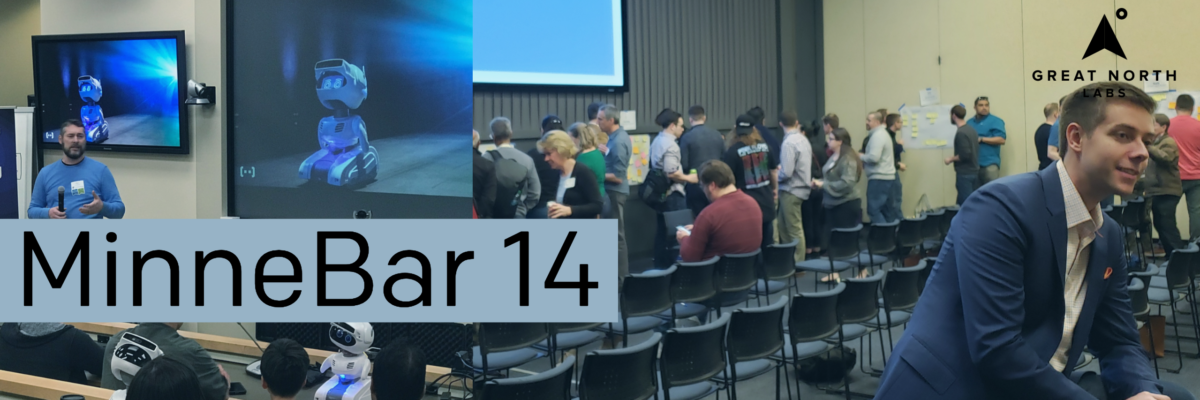
MinneBar 14 Recap
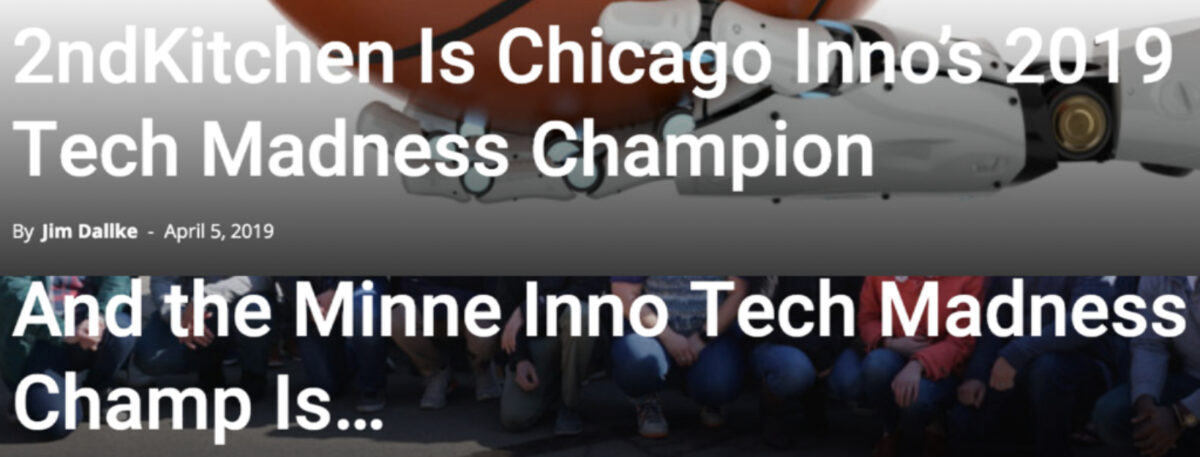
Dispatch and 2ndKitchen claim Tech Madness titles
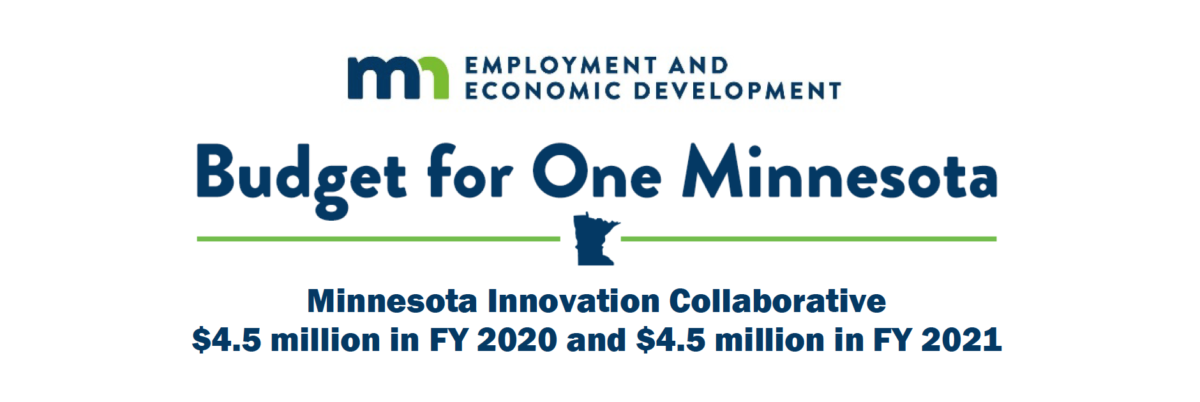
Minnesota Innovation Collaborative
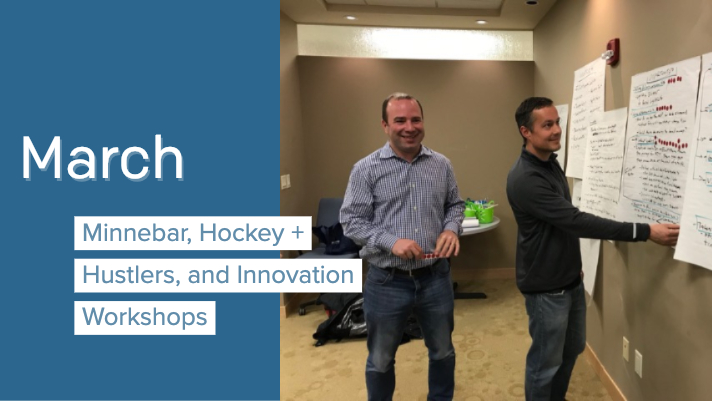
March: Minnebar, Hockey + Hustlers, and Innovation Workshops
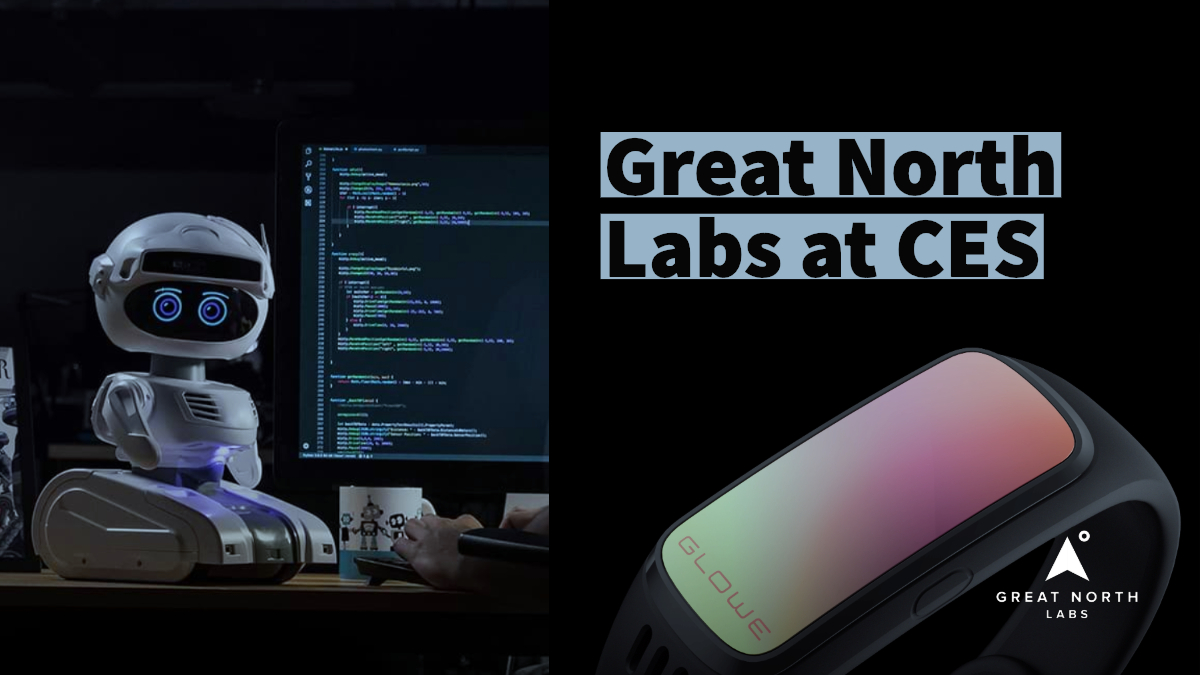
Great North Labs at CES
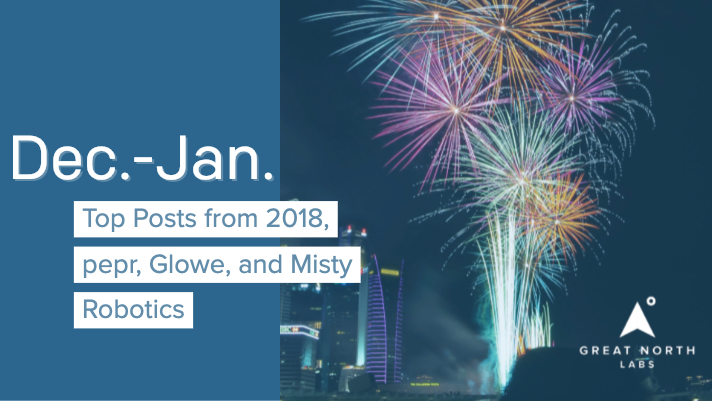
Dec.-Jan.: Top Posts from 2018, pepr, Glowe, and Misty Robotics
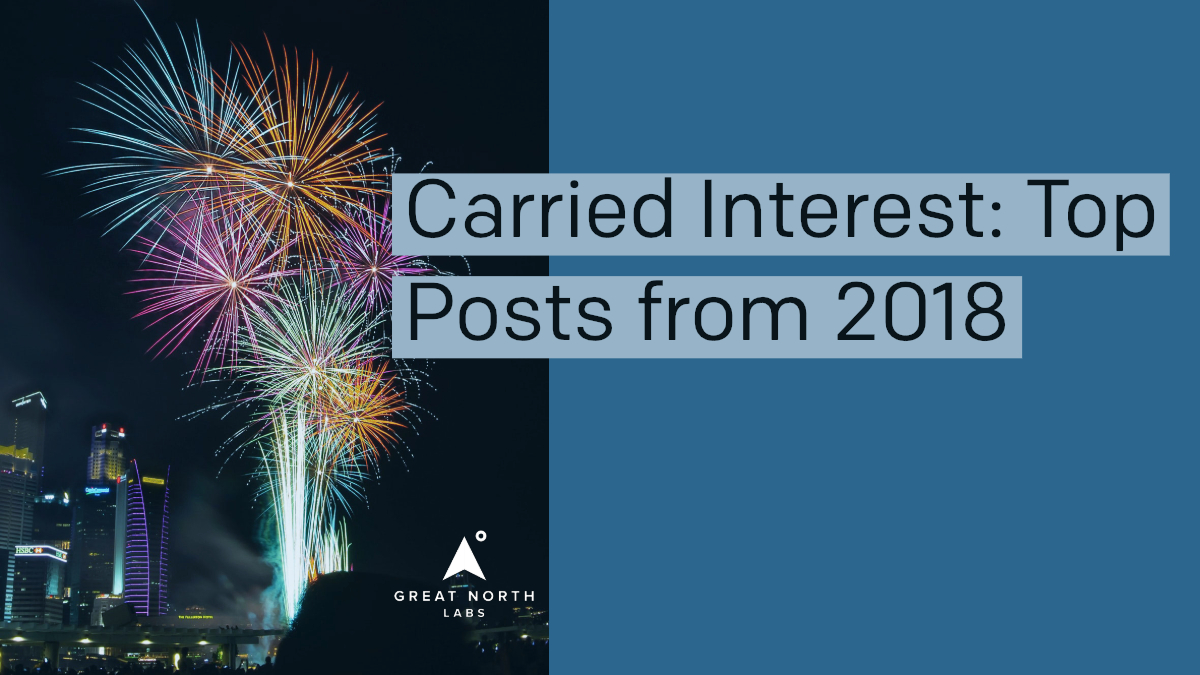
Carried Interest: Top Posts from 2018
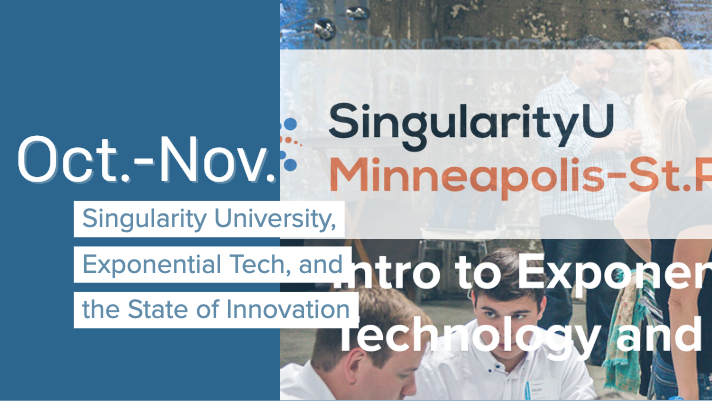
Oct.-Nov.: Singularity University, Exponential Tech, and the State of Innovation
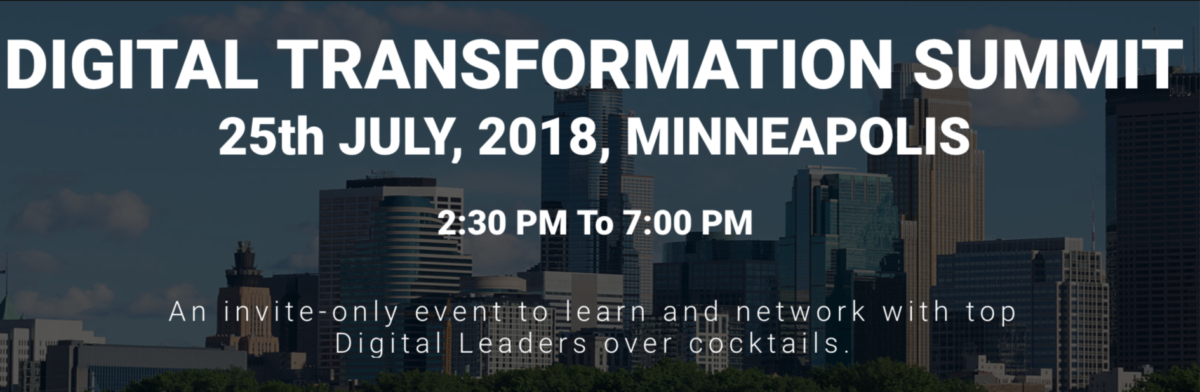
Digital Transformation Summit, July 25th in Minneapolis
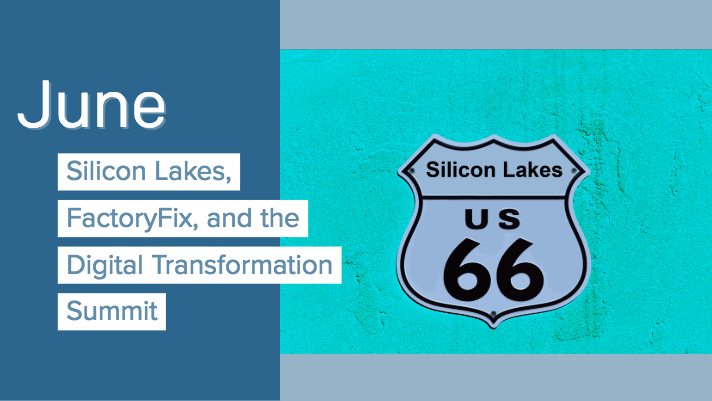
June: Silicon Lakes, FactoryFix, and the Digital Transformation Summit
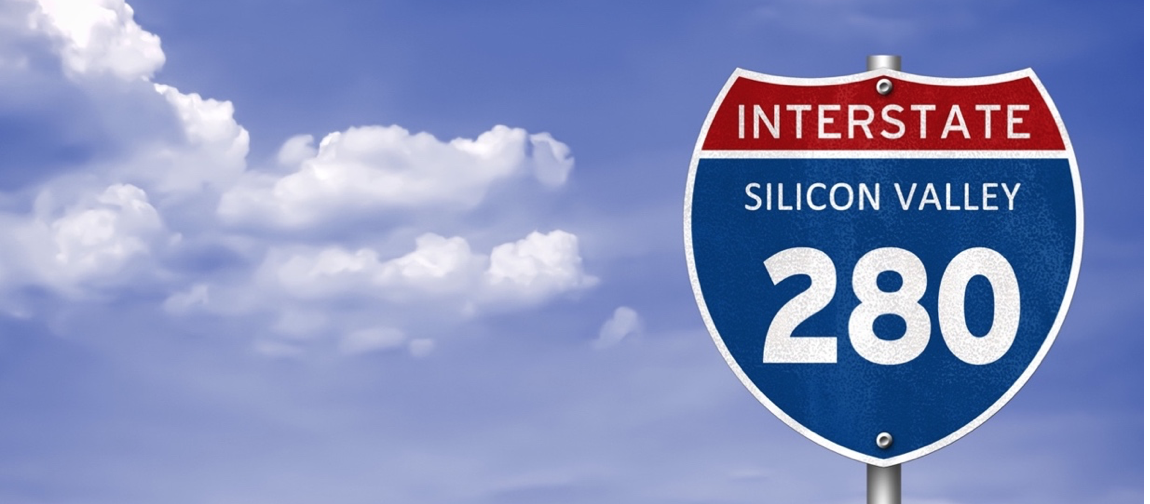
Putting the “Silicon” in Silicon Lakes
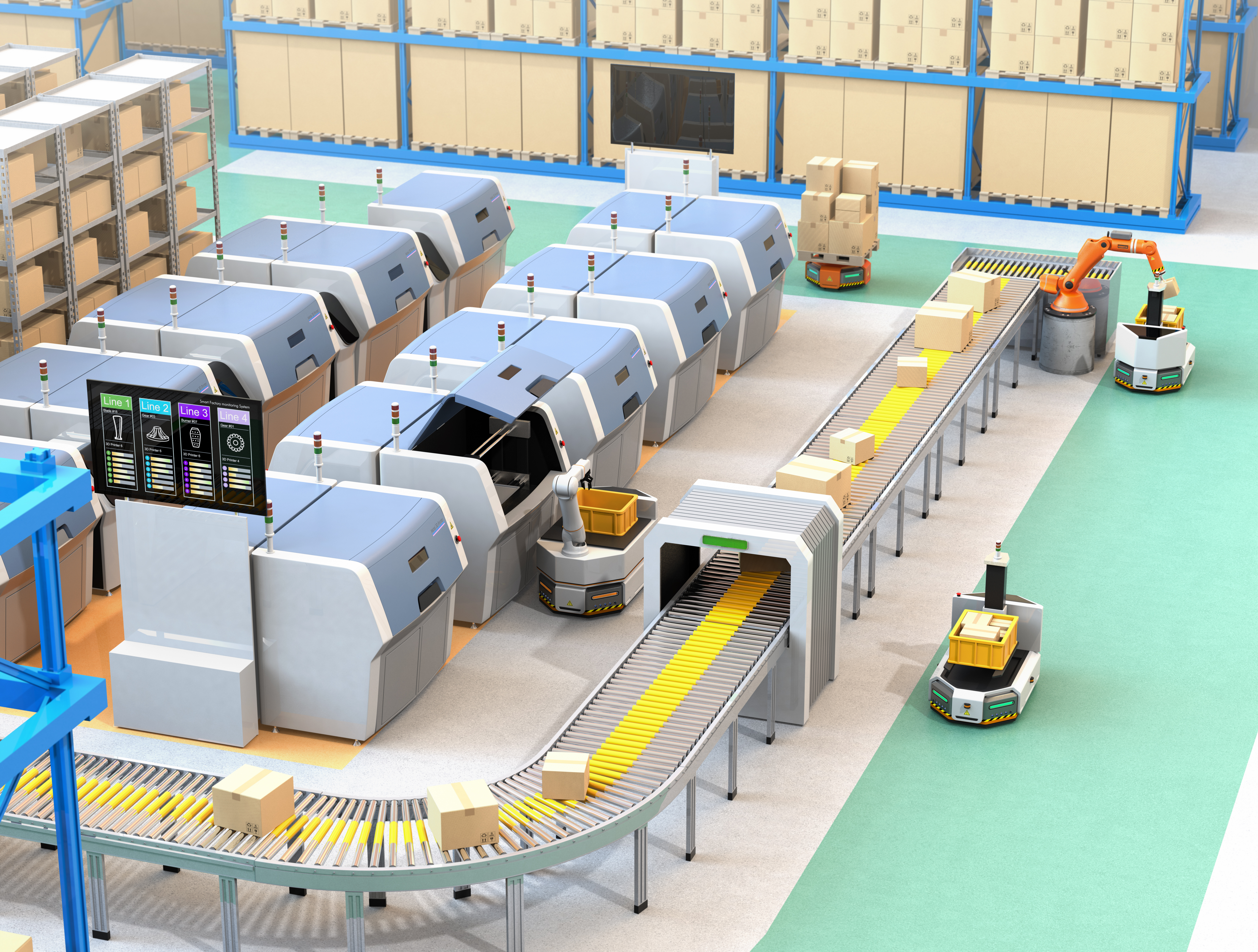
Digital Manufacturing and Logistics
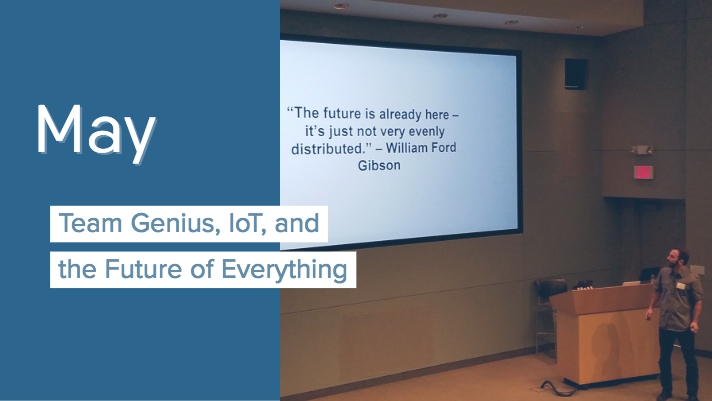
May: Team Genius, IoT, and the Future of Everything
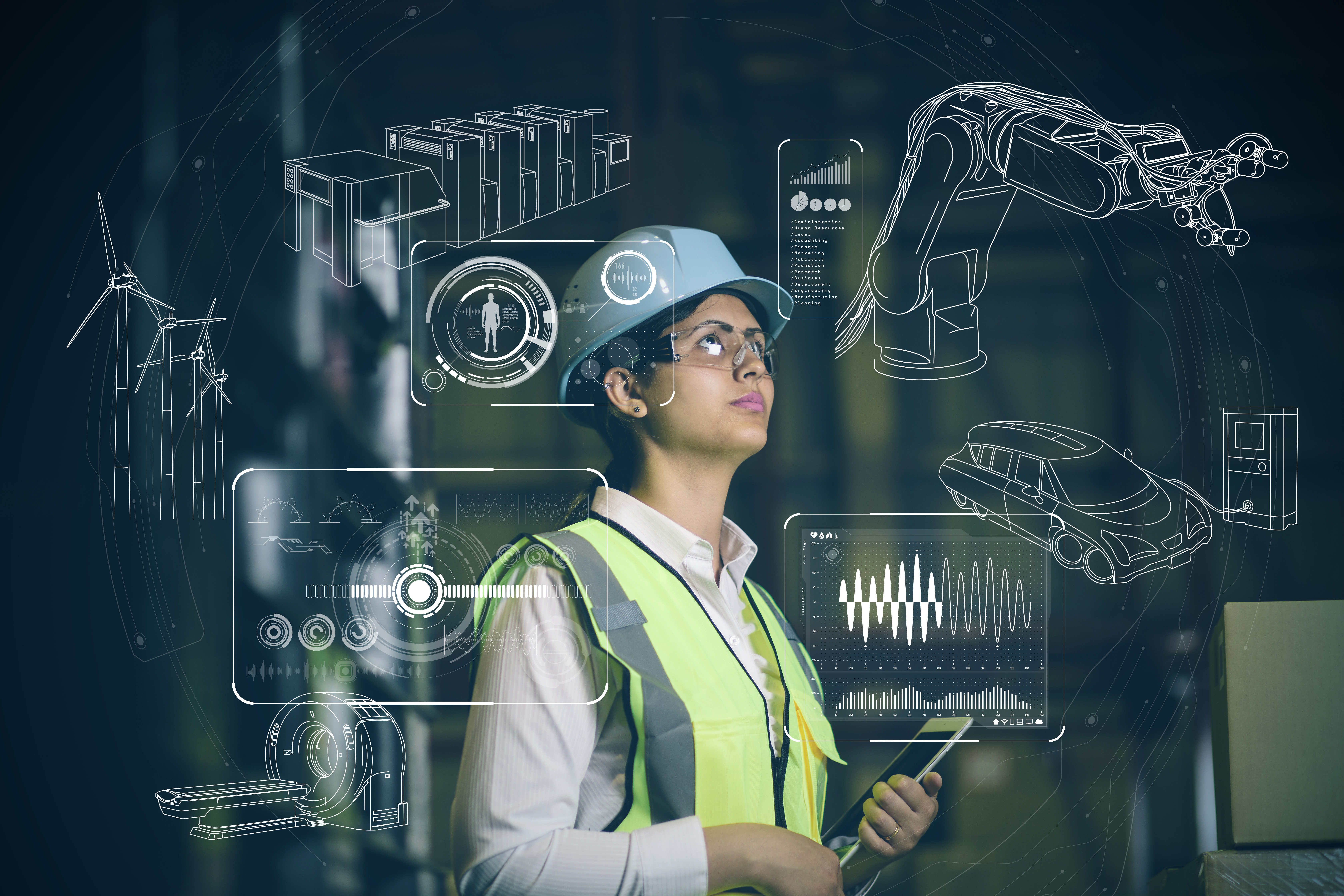
IoT 3.0
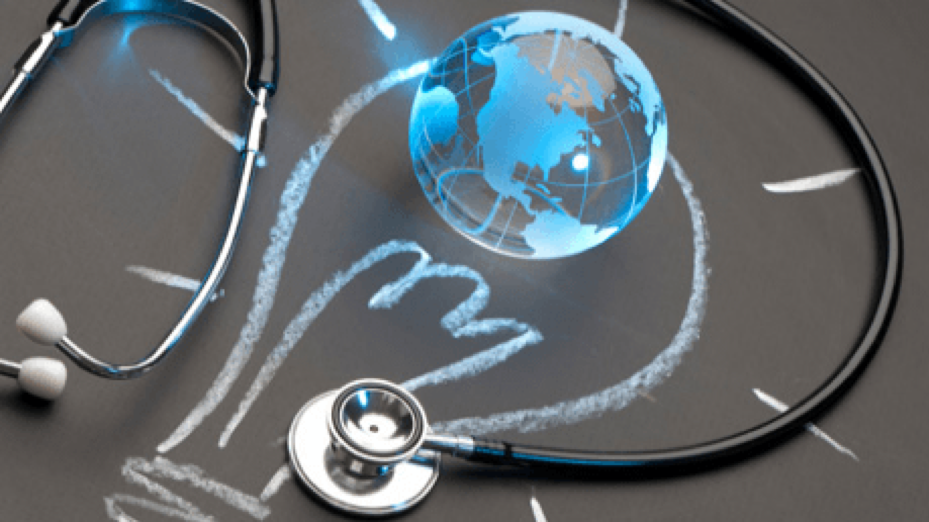
Healthcare Innovation
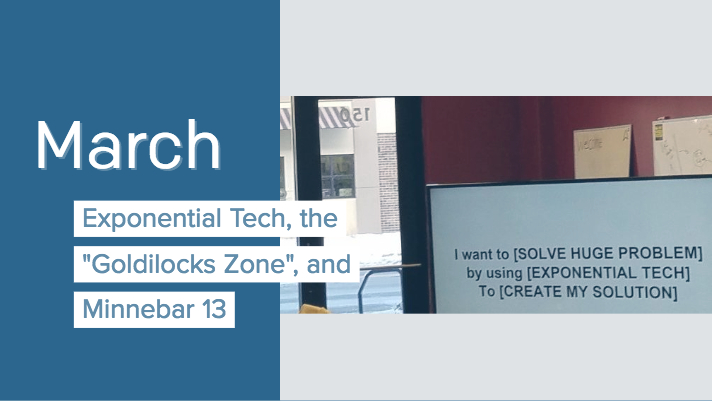
March: Exponential Tech, the “Goldilocks Zone”, and Minnebar 13
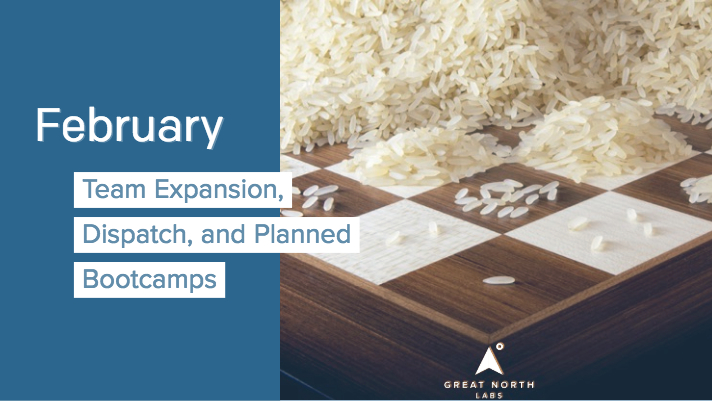
February: Team Expansion, Dispatch, and Startup School Events
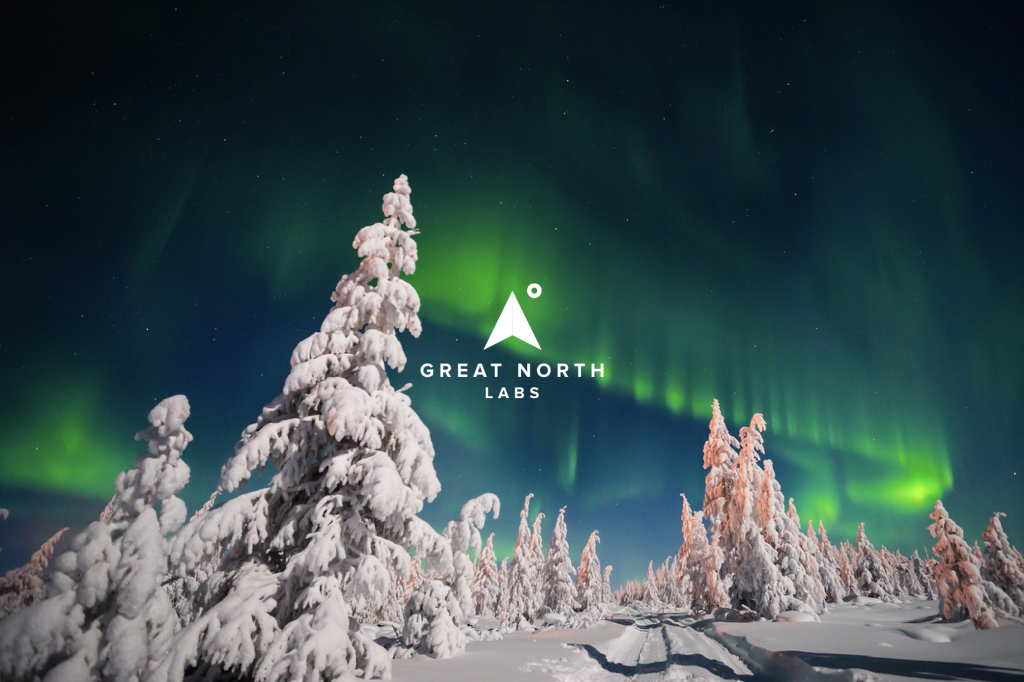
Great North Labs Newsletter – December 2017
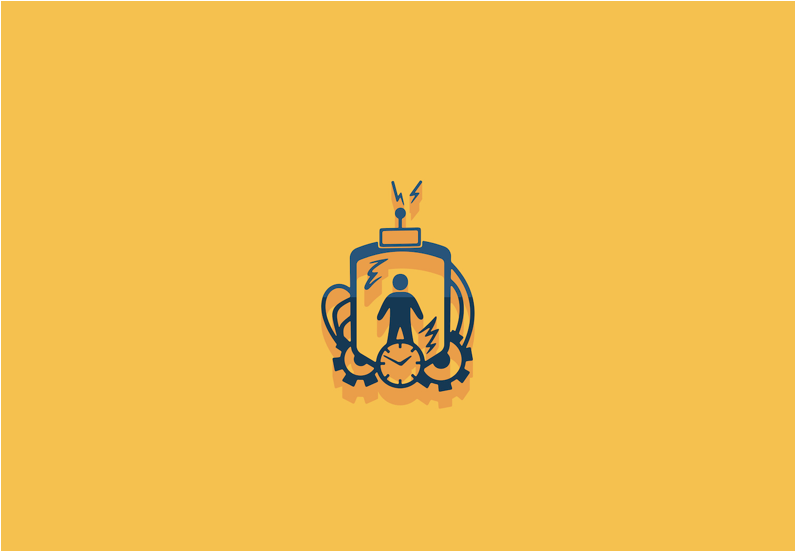
A Letter To My Younger Self
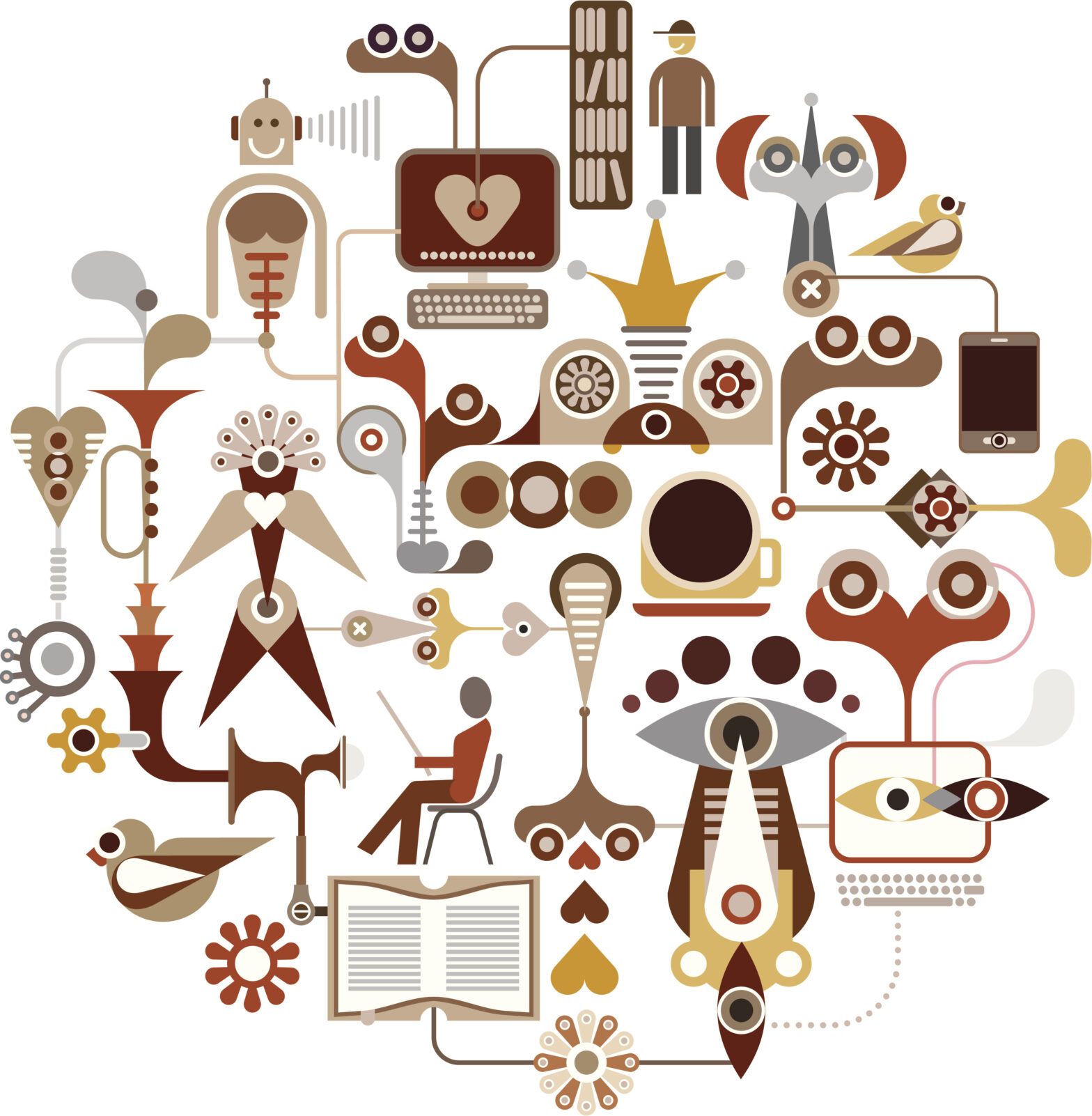
Great North Labs Newsletter
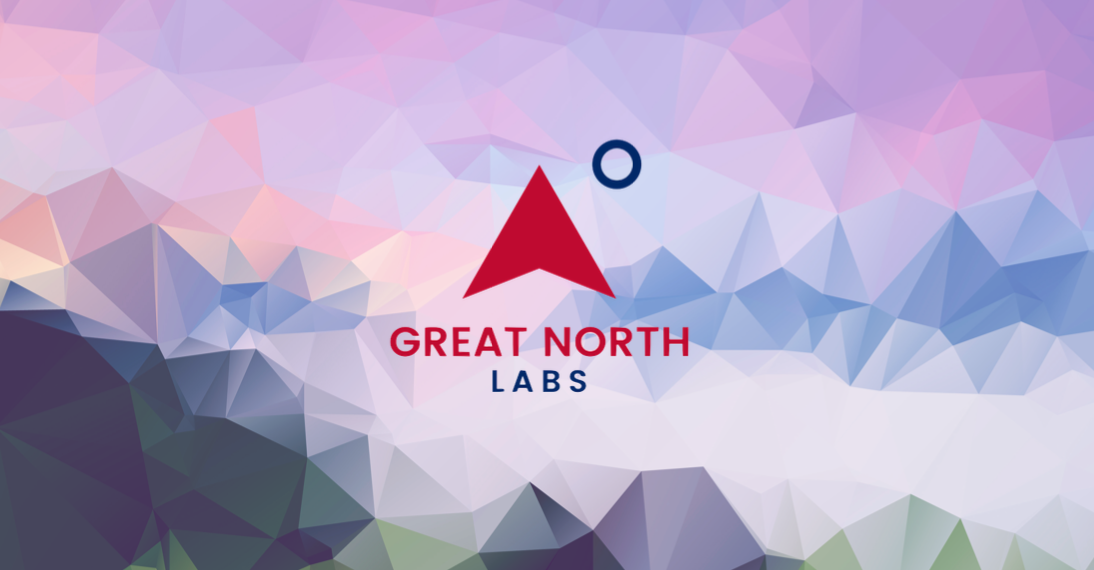
Great North Labs Featured on Tech.mn
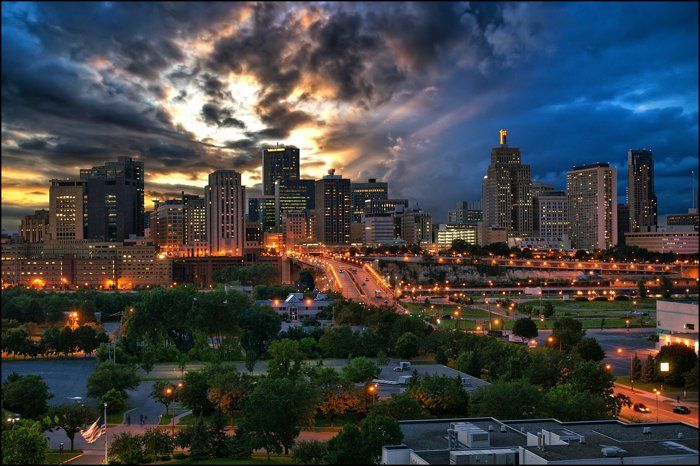
Great North Labs Featured in St Cloud Times
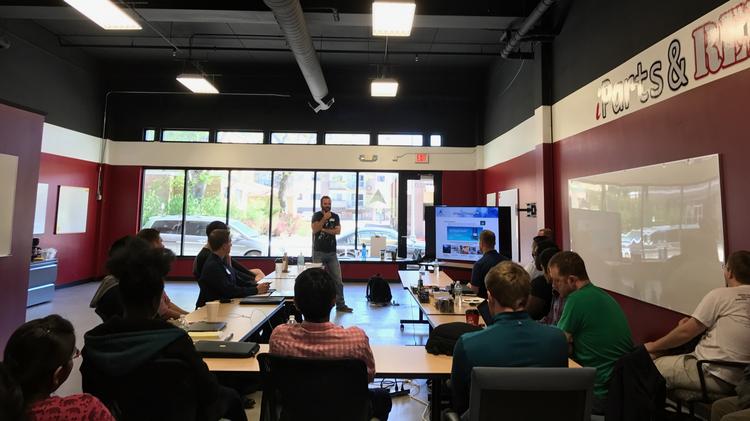
Great North Labs – Featured on BizJournals.com
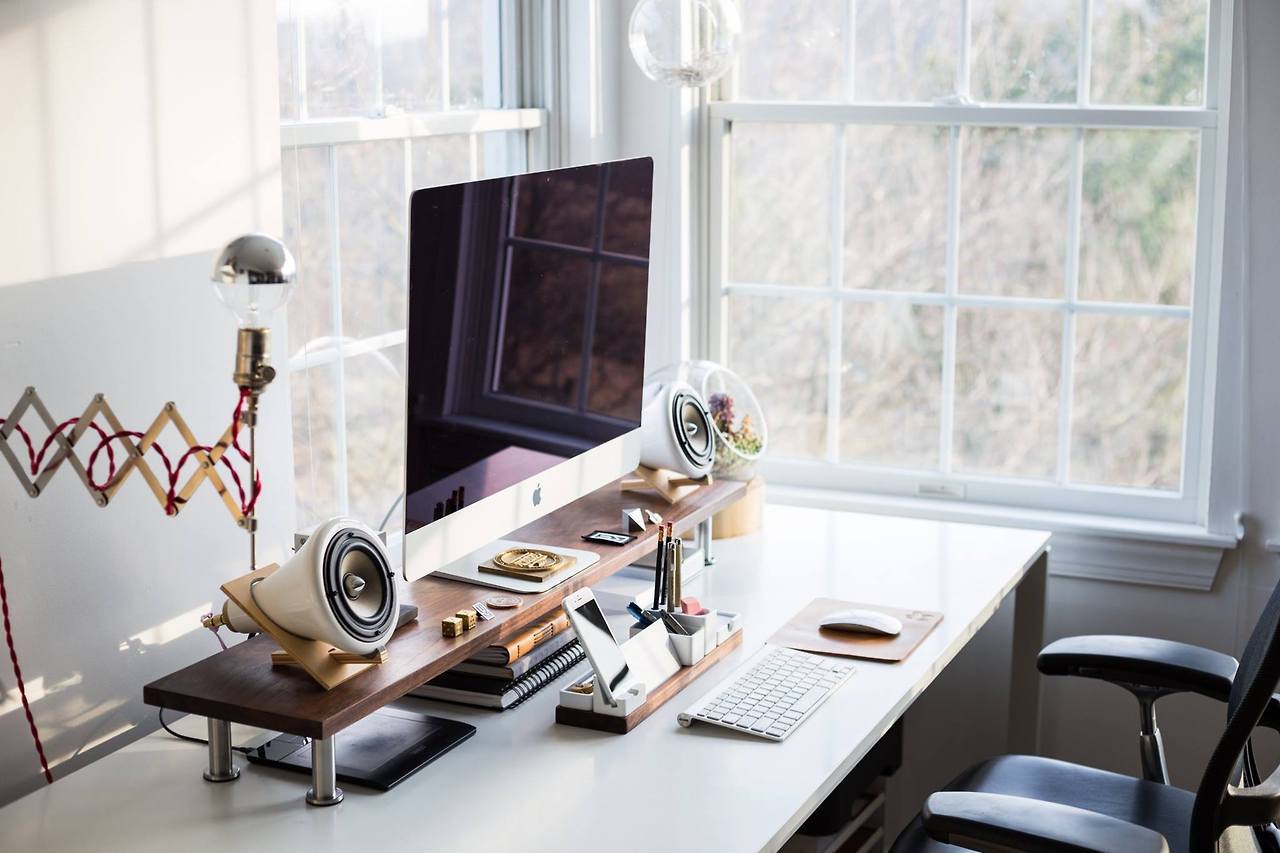